Cryptic Pockets: 'X' Marks the Spot
Cryptic binding pockets are like buried treasure. They're mysterious, difficult to find, and come with untold riches once surfaced.
Over 90% of FDA-approved drugs target proteins. The majority of these are small molecules that bind to inhibit a protein's function.
Some proteins, like enzymes, have well-defined grooves on their surfaces known as binding pockets. Enzymes catalyze chemical reactions within these binding pockets. If a drug developer wants to inhibit this catalytic function, they can design a small molecule (ligand) that snaps into the binding pocket.
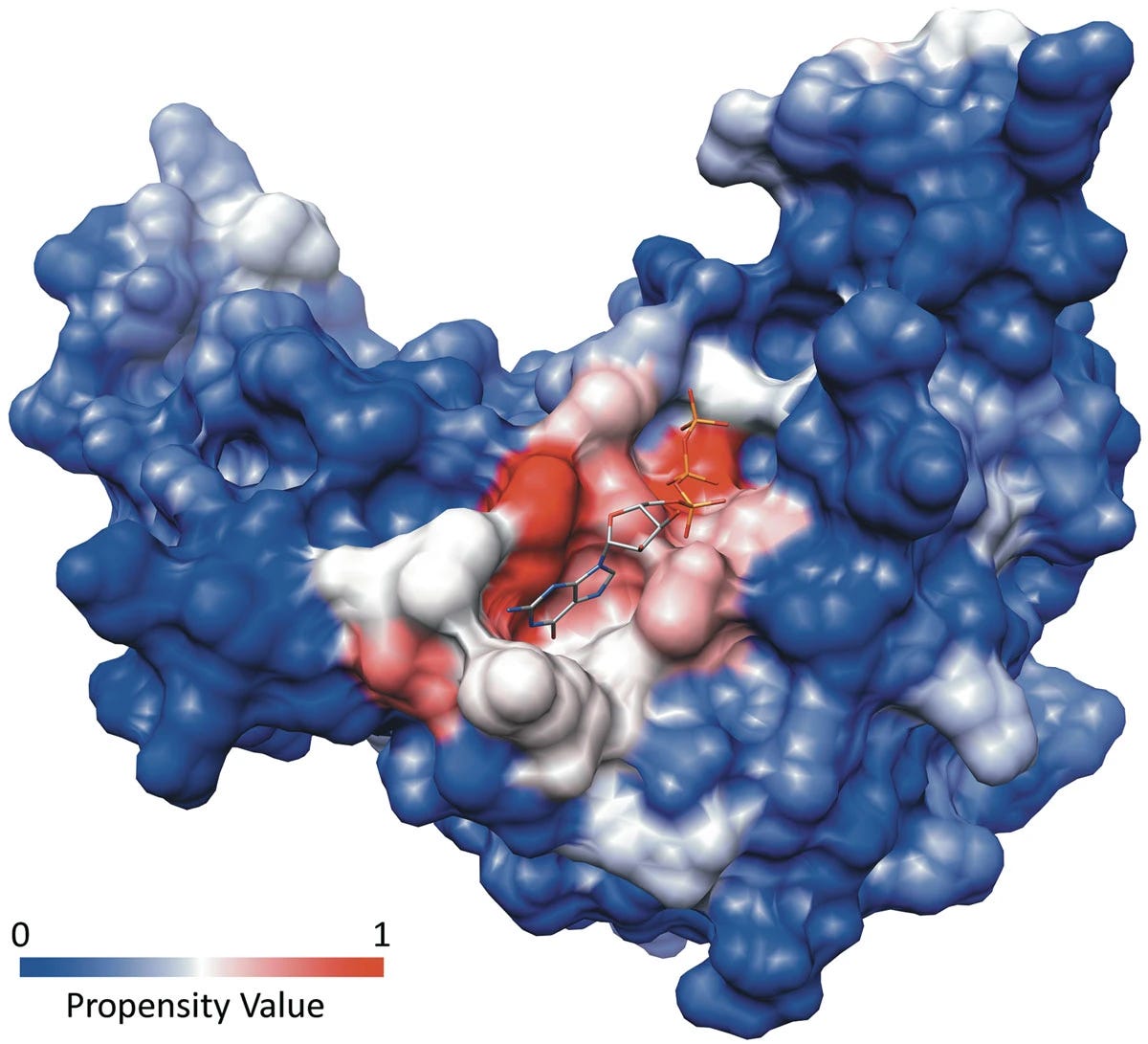
Not all proteins are as straightforward to drug. Transcription factors, for example, have smooth surfaces devoid of obvious binding pockets. Scaffolding proteins that form large protein-protein interactions (PPIs) typically lack so-called canonical binding sites.
Remember that proteins aren't static objects. They vibrate, twist, and contort through time, especially in response to the molecules around them. Canonical binding pockets persist throughout time and molecular contortions. You might wonder if there are non-canonical pockets, ones that exist rarely or that require neighboring molecules to tease out.
Rare, transient binding cavities are known as cryptic pockets.
The definition of a cryptic pocket is somewhat fluid. Generally, cryptic sites aren't easily detectable in a protein's unbound, ligand-free state. When ligands or nearby molecular shrapnel binds a protein, they can cause a conformational (structural) change that exposes cryptic binding sites. You can imagine it like a small key that opens a protein's secret compartment.
Why do drug developers care about targeting cryptic pockets?
More Treatable Diseases: The universe of proteins with canonical binding pockets is vastly smaller than that of proteins with cryptic pockets. Hitting cryptic sites broadens the landscape of druggable proteins, and thus, treatable diseases.
Less Toxic Drugs: Canonical binding sites are evolutionarily conserved. Related proteins have structurally similar binding pockets. This increases the likelihood that small molecules may erroneously bind to a similar, non-target protein. Cryptic sites are less conserved, making it easier to inhibit only the target protein and lessening off-target toxicity.
Overcoming Drug Resistance: Tumors have a nasty habit of mutating proteins in response to therapeutic pressure. Tumors sometimes alter proteins by changing or deleting amino acids that form canonical binding sites, effectively blocking inhibitors from continuing to work. By targeting cryptic pockets, inhibitors may be more likely to overcome common drug resistance mechanisms.
How do scientists discover cryptic pockets?
Targeting a protein's cryptic binding pockets has some obvious benefits, but the process of elucidating these hidden cavities is challenging. Molecular dynamics (MD) methods that model how proteins evolve through time are apt tools to discover transient, cryptic binding pockets.
The big issue is that cryptic pockets don't spontaneously appear. Generally, cryptic pockets are hydrophobic. They hate water and don't like being exposed to it. It requires large, often unfavorable, energetic swings in the form of large conformational changes to expose cryptic pockets.
Unbiased MD simulations are expensive and difficult to run for long time periods. Therefore, it's rare that these simulations will sample from energetically unfavorable regions of conformational space. It requires some clever trickery to bias MD simulations to selectively sample regions of the energy landscape that may contain cryptic binding sites.
There are a variety of ways to bias an MD simulation to explore valuable, rare events. One recent method is SWISH-X. Sampling Water Interfaces through Scaled Hamiltonians (SWISH) was the original method, which I'll describe first.
SWISH belongs to a family of MD methods that use a technique called replica exchange. Multiple copies (replicas) of a biochemical system are simulated in parallel, each with a slightly different Hamiltonian (energy function). In English, that means that the physical laws for each replicate are tweaked a bit. Periodically, the simulations are swapped, allowing the system to overcome larger energy barriers to explore novel regions of conformational space.
This can all be a bit fuzzy, so let's use an analogy.
Imagine there are many parallel versions (replicas) of you that exist in different timelines (simulations). You start at the bottom of the Grand Canyon and are tasked with finding a buried treasure (cryptic pocket) up on the desert surface. The steep, vertical facade of the canyon represents a large energy barrier.
If we had just one version of you, unaided at the bottom of the valley (unbiased MD), it's highly unlikely you'd be able to clamber up the rock face to find the treasure.
However, in our replica exchange version, we're free to alter the laws of physics in an unrealistic (alchemical) way. Let's say that we're varying the constant of gravity. For some replicas, gravity is reduced, allowing you to easily float up and out of the Grand Canyon and onto the surface. Suddenly, that version of you swaps (exchanges) timelines into a dimension with normal Earth gravity, freeing you to traverse the desert sands in search of treasure.
That's how replica exchange works in a nutshell.
Instead of gravity, SWISH alters the level of attraction between hydrophobic amino acids in a protein and the surrounding water molecules. By exaggerating this attraction, water essentially pries open cryptic pockets momentarily. When the replicas switch, neighboring organic molecules (often hydrophobic) can stabilize these cryptic pockets, keeping them open long enough to be more rigorously characterized. Treasure found!
If that's SWISH, then what is SWISH-X?
SWISH might work when the valley you need to climb out of is just the Grand Canyon. But what about if you need to float up the Marianas Trench, which is ~6x deeper?
The 'X' in SWISH-X stands for eXtended. With this method, the authors add in a second scaling factor: temperature. Specifically, they leverage a module called OPES MultiThermal which varies the temperature that each replica is run in alongside the water-attraction factor we discussed earlier.
Increasing temperature helps the replicas jump out of even deeper valleys. Hotter simulation conditions cause increased thermal fluctuations, meaning atoms vibrate more intensely, which can lead to larger-scale conformational changes and an increased likelihood of exposing a cryptic pocket. It would be like if we lowered gravity AND increased your buoyancy simultaneously to climb out of the murky depths of the Marianas.
How does SWISH-X perform?
It performs beautifully when measured up against other approaches. I'll walk through the violin plot below to explain what's going on.
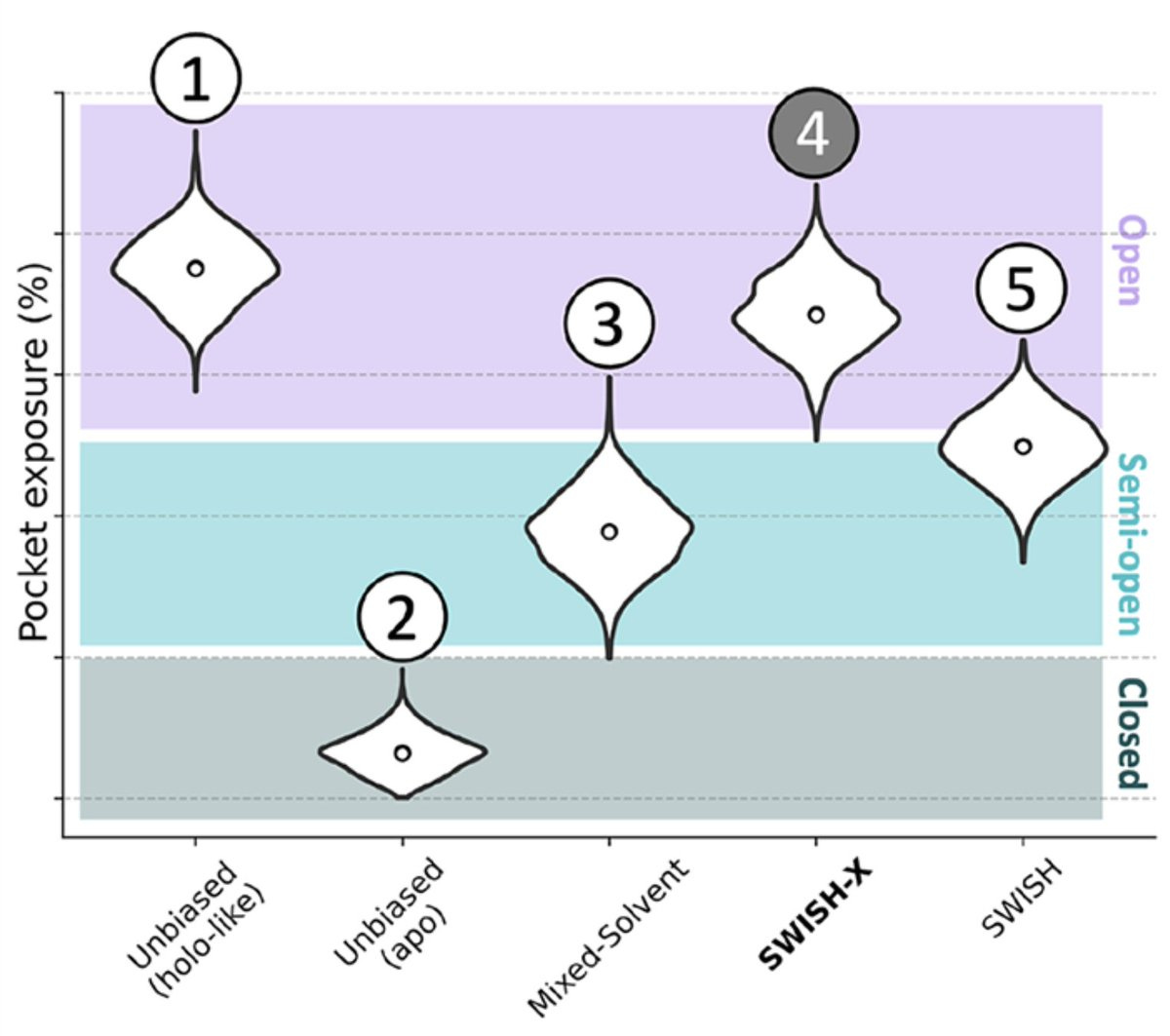
Let's start with the vertical axis. It's divided into three regions depending on the level of cryptic pocket exposure. The purple means the cryptic pocket has been discovered. Light blue means it's been partially characterized. Dark teal means we couldn't find it. In other words, better discovery methods should skew data towards the top of the plot.
The horizontal axis tracks various methods, including the left-most method which is the benchmark. Briefly, holo- structures are when the protein is bound to a ligand (inhibitor) whereas apo- structures just include the protein by itself, as shown below. Remember earlier when I said that cryptic pockets tend to expose themselves when bound to a ligand? Well, that's what we're comparing to on the left: the perfectly open (essentially solved) structure that is 'holo-like'. The closer our data looks to that, the better.
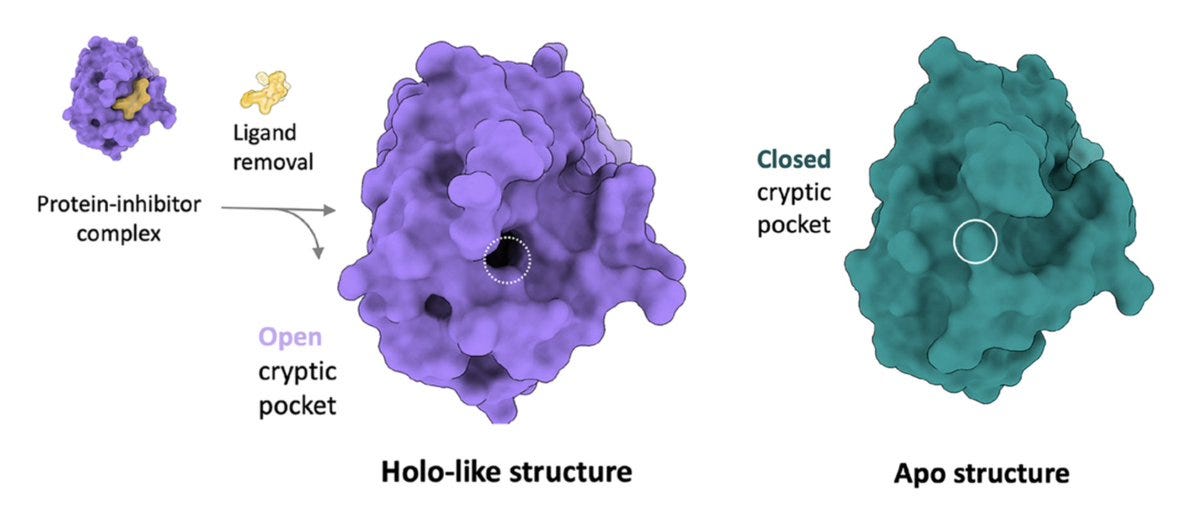
Doing unbiased MD on the apo- structure is awful, like climbing out of the Grand Canyon under Jupiter-like gravity and without a rope. Using a mixed-solvent approach, a simulation with explicit neighboring water and organic molecules is better. These can bind the protein and induce partial cryptic pocket opening, like we discussed earlier.
SWISH is even better yet! Half of the distribution results in an open, characterized pocket. Finally, SWISH-X performs the best with virtually all of its distribution resulting in a solved cryptic pocket. SWISH-X marks the spot!
At Dimension, we are keenly focused on the interface of MD and machine learning (ML). If you're working at this interface or are scouring the Earth for buried cryptic pocket treasure, don't be a stranger!
If you'd like to hear more of what we have to say about the present-future of hybrid physics-ML methods in drug discovery, read our recent (open-access) journal article. Finally, if you want to read more about SWISH-X, check out the full publication!
Very informative post! You did a good job explaining replica exchange. The analogy of multiple parallel universes, each of which has different physical laws, was very effective. I especially liked your use of the word "alchemical" to describe these deviations from physical constraints.
If I correctly understand the figure comparing different techniques for cryptic pocket discovery, it's saying that SWISH-X is successful at identifying confirmed cryptic pockets. However, how effective is this method for identifying *novel* pockets? Considering that the replica exchange approach involves deviating from physical laws, I would expect a fairly high rate of false positives. Has this been estimated?
Fascinating work. I'm curious if organisms that persist in several distinct environments over their life (like parasites) have proteins that exhibit more cryptic switches? A tool like SWISHX will be invaluable for systematically testing these kinds of hypotheses.