Novel Cancer Biomarkers Will Fuel Next-Generation Trial Design
Researchers are gaining a dynamic lens into a tumor's molecular response to therapeutic pressure. As these data are combined with modern ML, how might cancer trial design evolve over this decade?
The last decade has seen a historic outpouring of investment into the life sciences. Driven by a more laissez faire FDA, low interest rates, and thematic exuberance for precision medicine—a substantial amount of capital flowed into preclinical therapeutics companies. Fledgling biotechs cited the secular decay in the industry’s R&D efficiency (Eroom’s Law), claiming that emerging technologies, if properly amalgamated, could reverse the trend.
Pharma industry statistics have warped in response. Biotechs accounted for 70% of the global clinical development pipeline in 2021. Contract research organizations (CROs) estimate that biotechs’ annual R&D spend will double that of established pharma over the next several years. Since 2008, the volume of Phase 3 trials sponsored by biotechs has tripled. In 2021, biotechs were responsible for roughly two thirds of FDA approvals.
Clearly, emerging biotechs are building the front of the funnel and taking drugs through the finish line. The data on ‘cheaper, better, and faster’ hasn’t manifested yet, however. What I am certain of is that nascent biotechs seeking R&D efficiency Valhalla will need to innovate downstream within the clinical development phases. Tech-enabled, preclinical science engines can only do so much to stem clinical translation inefficiencies.
Decentralized clinical trials. Patient-centric design. Real world evidence. There are myriad clinical development efficiency trends. Though all are interesting, I find the intersection of novel biomarkers and adaptive clinical trial design particularly compelling. To that end, I think minimal residual disease (MRD) assays powering adaptive oncology trials could meaningfully improve solid tumor asset translation efficiency over the next market cycle.
The unequivocal gold-standard in clinical development is the randomized controlled trial (RCT). These fixed protocols involve a linear progression through design, execution, and analysis phases. Adaptive designs (ADs) are more flexible. ADs feature an iterative feedback loop, as shown below. This mechanism allows a sponsor to make pre-specified alterations to the trial based on interim data analyses. ADs aim to make trials more efficient while preserving the integrity of the underlying data.
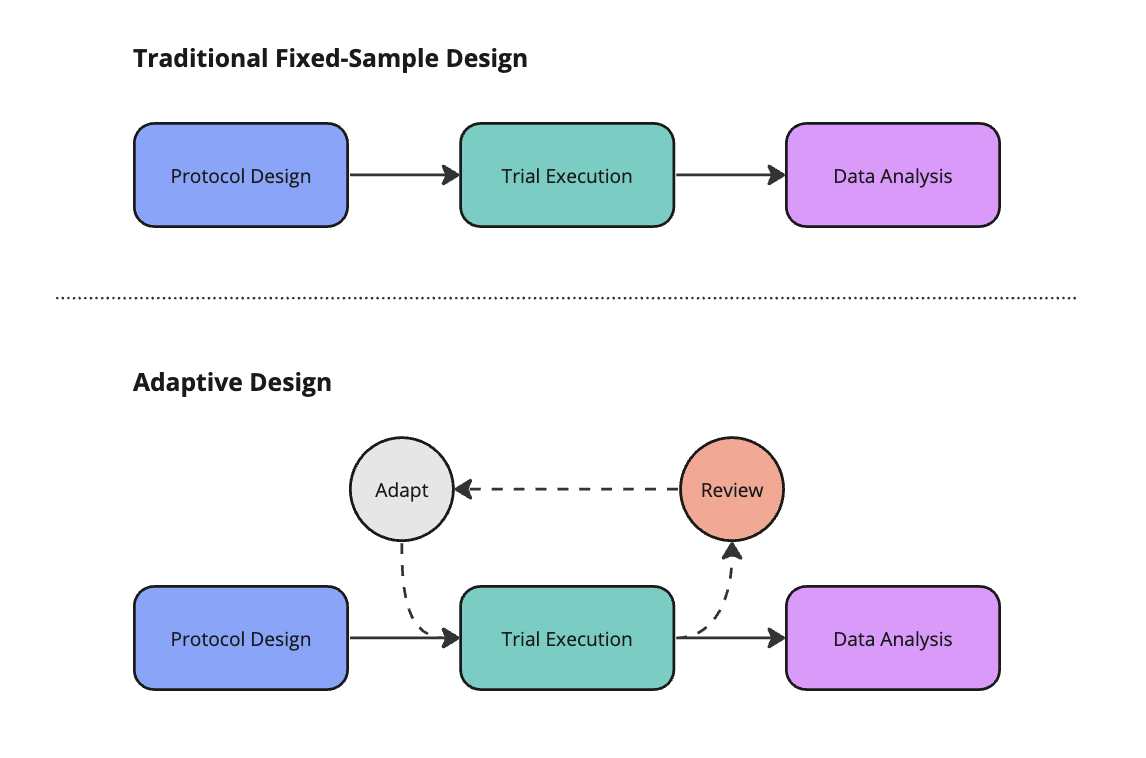
Well-designed AD require sponsors to enumerate potential adaptations before trial initiation. Common adaptations include changing the sample size, abandoning treatment or dose arms, modifying the allocation of patients randomized to different arms, enriching for a patient sub-population most likely to derive benefit, or ceasing the trial early on account of success or futility.
ADs aren’t new—they’ve been around for two decades. Despite their tenure and ostensible advantages, ADs are underutilized. Tufts found that ADs represented just 20% of new trial starts in 2014. Compared to RCTs, ADs can feature higher upfront costs, complicated protocol designs, and logistical challenges affecting their execution. The flexibility of ADs can sometimes be a bug rather than a feature.
The repertoire of potential adaptations as well as their aims, advantages and disadvantages, is daunting. Tracking these considerations and communicating them to all trial stakeholders is harder still. Taken together—these degrees of freedom may have diluted the perception of ADs, making them feel statistically unrigorous and full of ad-hoc changes. Some biotech sponsors fear the flexibility of ADs may invite significant regulatory scrutiny. Would all the effort be worth it then?
In November 2019, the FDA issued its first guidance document for implementing ADs. This clarified best practices for ADs and signaled the agency’s warming attitude towards ADs as an acceptable format. Despite the harrowing impact that COVID-19 had on global clinical trial enrollment, there were more ADs launched in 2020 alone than in the previous decade combined. As trial volumes continue to normalize, ADs are poised to continue taking share.
Sponsors must repeatedly collect high-quality data to make appropriate AD recommendations. Solid tumor trials are uniquely challenging. Patients can’t undergo tissue biopsies every month. Therefore, radiography has become the predominant tool to inform AD feedback loops.
Though well established in guidelines like RECIST, radiography has two key weaknesses for assessing solid tumor burden. First, imaging isn’t as sensitive as next-generation sequencing (NGS)-based approaches, creating a lag between molecular and radiographic relapse, as illustrated below. Second, imaging can’t collect molecular information about tumors.
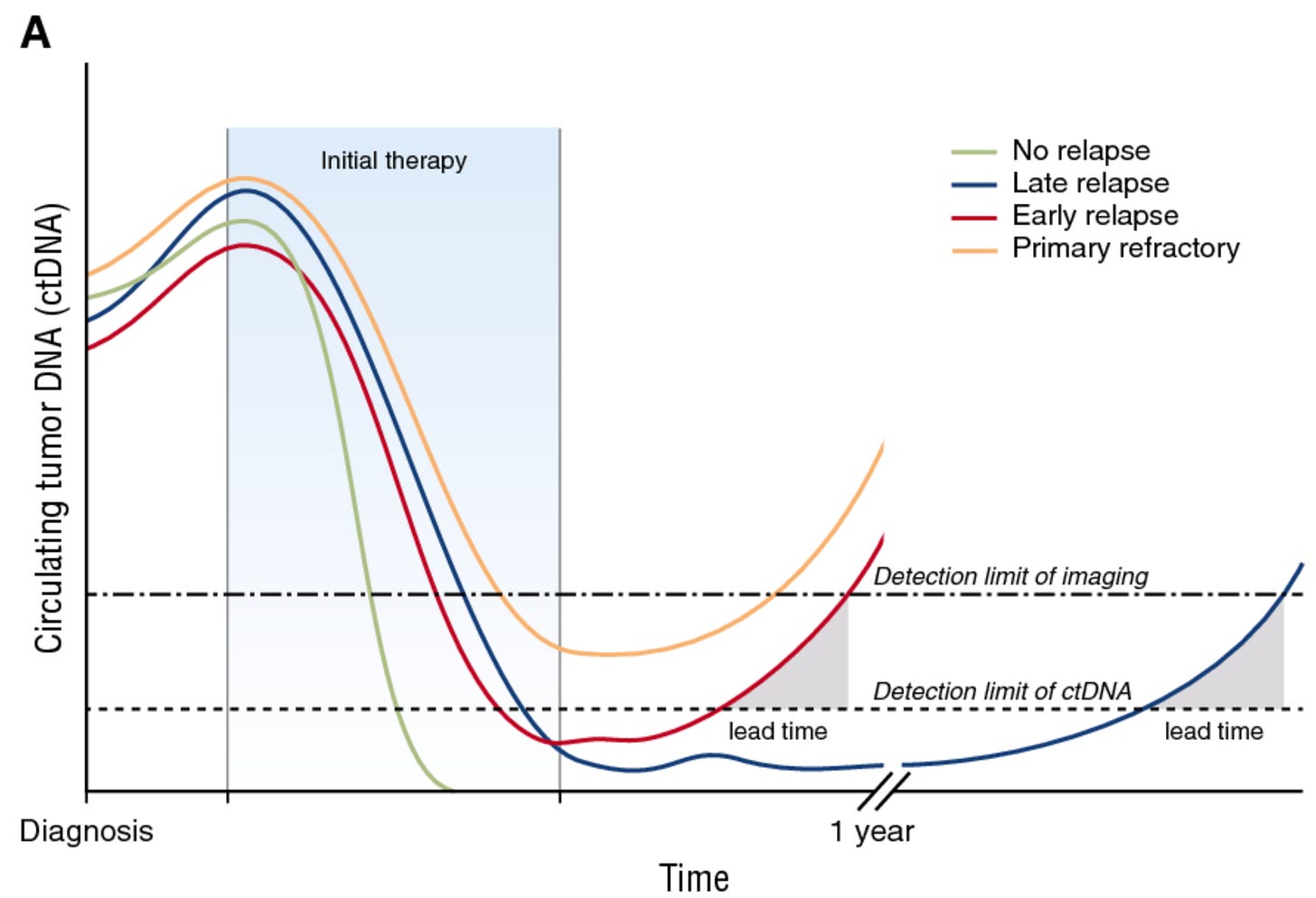
These and other shortcomings prompted the development of minimal residual disease (MRD) tests for solid cancers. MRD assays typically use NGS to analyze circulating tumor DNA (ctDNA) in a patient’s bloodstream. Legacy tests leveraging circulating tumor cells (CTCs) are not terribly sensitive. PCR-based tests are sensitive, but restricted to small panels of somatic mutations. Therefore, modern MRD tests capable of analyzing entire cancer genomes (and epigenomes) are novel instruments powering trial design.
Biopharma companies usually are early adopters of emerging molecular diagnostic assays. Though not exclusively an adaptive mechanism, several biopharma sponsors have used MRD status for cohort enrichment. The logic is that patients with high levels of ctDNA following primary treatment (e.g., surgery) are more likely to relapse. By enrolling patients more likely to recur, biopharmas investigating a second line intervention may be able to power efficacy endpoints with fewer patients—saving time and money.
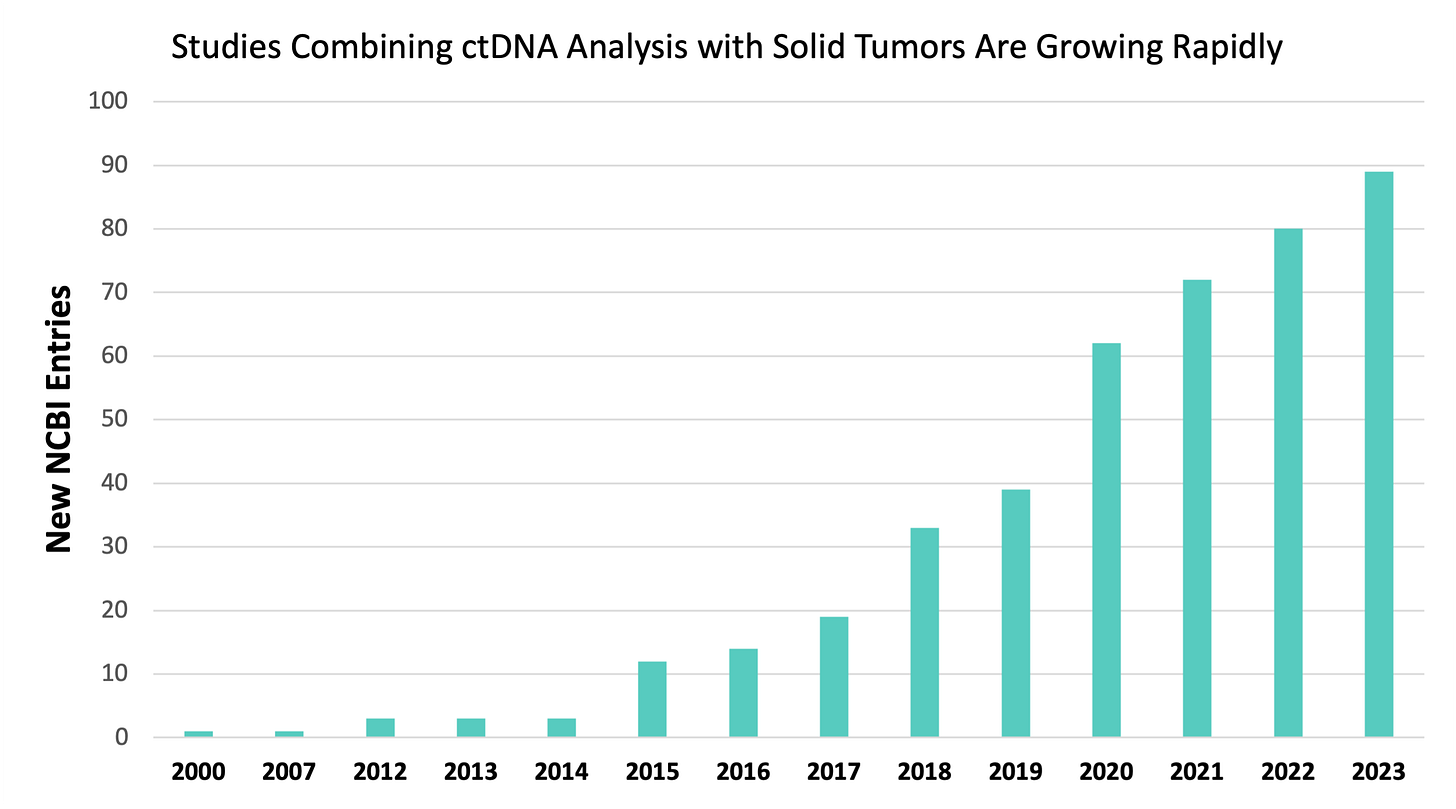
In 2022, the FDA released draft guidance on ctDNA-guided clinical trials. Cohort enrichment got a special shoutout. The agency opined on ctDNA as a measure of clinical response and an early (surrogate) endpoint—both having implications for AD trials. Sponsors theoretically could use ctDNA-derived biomarkers to alter inclusion/exclusion criteria, adjust allocation ratios between trial arms, or end a trial arm early for efficacy/futility. There’s still a vast evidence and implementation gap between the here and there—more on this later.
I’m excited by ctDNA kinetics as a biomarker for designing adaptive trials. Kinetics refers to the change (delta) between ctDNA levels at various timepoints. Once a baseline is established, ctDNA kinetics correlate well to changes in tumor burden—perhaps even better than radiographic measures. Authors at the Princess Margaret Cancer Centre recently compiled characteristic ctDNA dynamics following a range of standard interventions including surgery, radiotherapy, chemotherapy, and immunotherapy. The researchers highlighted AD strategies built around these interventions and ctDNA kinetics, illustrating the breadth of kinetic readouts in adaptive trials.
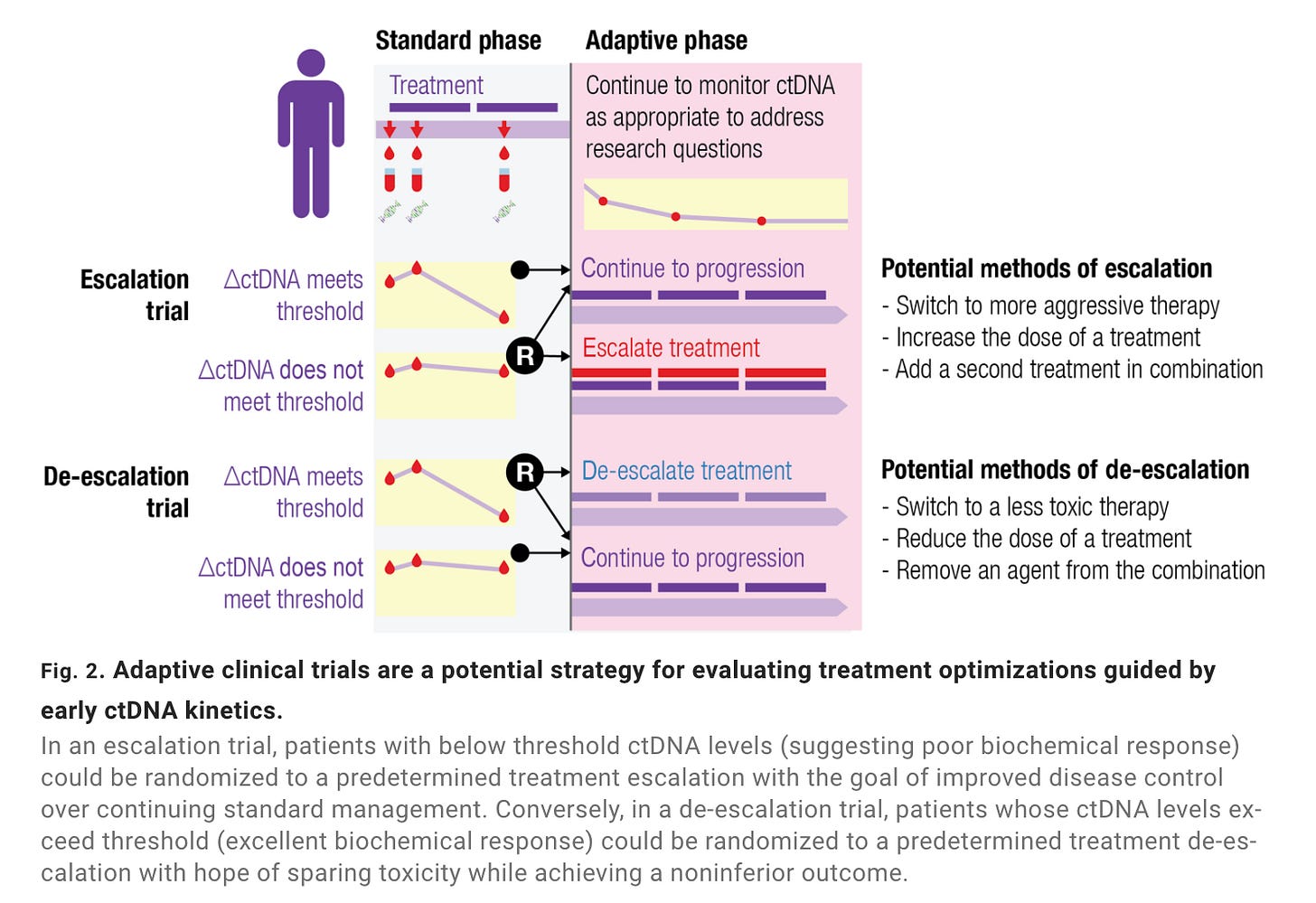
Jointly sponsored by Johns Hopkins and the Canadian Cancer Trials Group, BR.36 is an adaptive Phase 2 trial in lung cancer (NSCLC) leveraging ctDNA kinetics following treatment with standard-of-care (SOC) pembrolizumab. Though limited in power, the study suggested ctDNA kinetics were predictive of standard radiographic (RECIST) evidence (AUC = 0.77). The authors also showed how ctDNA response correlated to progression-free survival (PFS) and overall survival (OS), though these were underpowered (secondary) endpoints, as shown below. These results aren’t dissimilar from the recent DYNAMIC trial (n=455) in colorectal cancer (CRC) that assessed ctDNA status as a predictor of recurrence free survival.
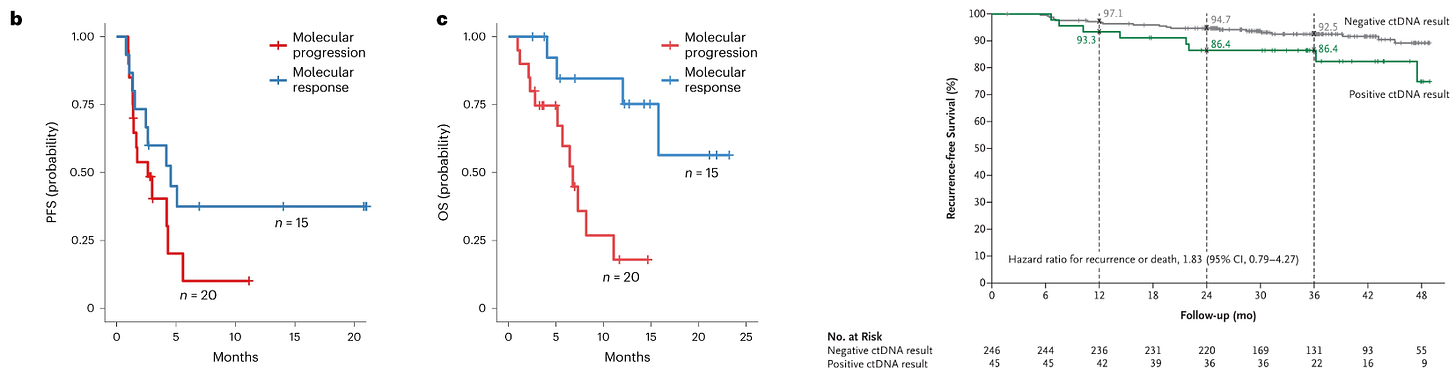
The second half of BR.36 should enroll enough patients to further power these early signals. Importantly, the sponsors will adapt randomization to a treatment intensification or SOC arm depending on ctDNA response. Generating evidence around efficacy outcomes as guided by ctDNA is critical to cement MRD within adaptive design frameworks. It’s imperative to show that early interventions equate to improved survival beyond what could be an illusory lead-time bias.
Dissociating from a multi-decade decline in biotech R&D efficiency will require innovation across preclinical and clinical development. Today’s capital constrained regime will force tech-enabled biotechs to reimagine clinical trial design. Next-generation trial design, inclusive of ADs, could serve this growing demand. Making decisions based on incomplete data is core to ADs and also an area benefiting from secular growth in machine learning (ML) methods like reinforcement learning.
ML’s present-future role in clinical trial design is rapidly evolving. I’ll save a thorough discussion for another article. However, I estimate ML will manifest first in early-stage trials (e.g., dose finding) across protocol design, logistics, patient recruitment, and other functions. My current favorite lab to watch in this space is the van der Schaar lab, which offers a wellspring of information about ML and next-generation trial design.
Like all data-driven techniques, ML improves monotonically with better data. Oncology AD trials demand better data. Though ubiquitous, imaging is a crude instrument to dynamically assess cancer’s response to therapeutic pressure. Radiography is less sensitive and lower fidelity than emerging approaches based on NGS. Alongside standard clinical data, ctDNA-based assays provide a high-resolution data stream that is low latency and increasingly backed by clinical evidence.
Should the evidence read positively, MRD is poised to become a dominant surveillance tool for oncology AD trials. NGS-based assays align well with a major secular shift we’re seeing with precision oncology drugs—they’re increasingly targeting specific genetic mutations. Solid tumors are an amalgam of many genetically-defined sub-diseases masquerading as a single disease. Unlike imaging, modern MRD assays give researchers a dynamic view into this heterogeneity.
Broad ctDNA assays have an enormous headroom for future clinical trial design objectives, especially for emerging therapies. For example, Relay’s forthcoming allosteric inhibitor of PI3K-alpha (RLY-2608) seeks to overcome the issue of adaptive resistance—a phenomenon where orthosteric inhibitors lose efficacy as a tumor mutates the protein’s active site. Using ctDNA MRD assays, the sponsor could prove the PI3K-alpha mutated clone responded despite acquired active site mutations.
Despite our optimism around ctDNA, we’re also following parallel tracks wherein AI is improving other modalities relevant to oncology. These include extracting ‘omics data from industry-standard H&E slides and ML applied to imaging data, for example. As we wrote in our letter to our future entrepreneurs, Dimension is steadfastly committed to empowering ideas at the interface of technology and the life sciences. In practice, this means everything from early pre-clinical assays or enabling infrastructure to commercial pipeline strategy and clinical development tools. If you’re working in or around this intersection, you should feel welcomed to reach out to us.
—Simon Barnett, Head of Research
I'm really excited that adaptive trial design is finally getting noticed instead of being a niche topic that only elite cancer trialists get excited about.
Have you seen the new program from ARPA-H?
https://arpa-h.gov/research-and-funding/programs/adapt
They hope to spur development of adaptive biomarkers for evolving metastatic cancers with the kind of trial design that ought to be the gold standard for precision medicine.
Really enjoyed reading + love the clinical context of the ctDNA seq advancement.