Old Dogs, New Tricks: Molecular Dynamics can Enhance Protein Degrader Design
Though not discussed as much as machine learning (ML) methods, molecular dynamics are a critical pillar of modern drug design and may hold additional advantages for newer modalities like degraders.
Molecular dynamics (MD) simulations have proven foundational to the development of traditional small molecule drugs (TSMDs). Drug developers use MD to simulate how proteins, TSMDs, and background atoms influence each other to move. Because biology is in constant motion, rigorous MD simulations help scientists overcome the limitations of static modeling techniques.
Rooted in physics, MD is applicable to any arbitrary system of atoms unlike machine learning (ML) methods that require sufficiently large, representative corpora of data for training. Given the similarities between TSMDs and heterobifunctional protein degraders (e.g., PROTACs), it’s no wonder that scientists have begun extending MD to the problem of degrader design. We argue that the unique pharmacology of PROTACs combined with their larger average size and lack of public training data make MD an especially vital tool for drug development.
Let’s start by reviewing PROTAC structure. As shown below, these tripartite molecules are composed of two protein-binding ligands connected by a linker motif. One ligand (the warhead) binds the target protein of interest (POI) while the other recruits an E3 ligase—a key member of a cell’s protein degradation machinery. Given their similarity to TSMDs, PROTAC ligands stand to benefit more readily from existing design tools.
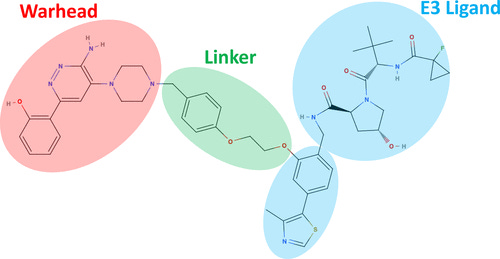
Linkers are more novel to PROTACs and are critically important for optimizing degradation efficiency. Several groups have nominated generative algorithms as well as benchmarks for evaluating linker generation—though it’s still early days. Compared to TSMDs, synthetic linker libraries and ground-truth data on linkers’ effects on degradation are sparse. We do know, however, that linker geometry and flexibility impart a material effect on the stability of the complex formed by the POI and E3 ligase. While simulating PROTAC molecules themselves is crucial, a key application area for MD is modeling the interactions between the POI and E3 ligase.
Unlike TSMDs, heterobifunctional molecules create a ternary complex involving two proteins and the drug itself. Protein complexes are not static structures—they exist as a translucent fabric of probability-weighted conformations. They prefer to live in low free energy conformations. Benefitting from eons of co-evolution, complexes like hemoglobin have deep conformational free energy landscapes, ensuring they fold up consistently and functionally, as shown below (left).
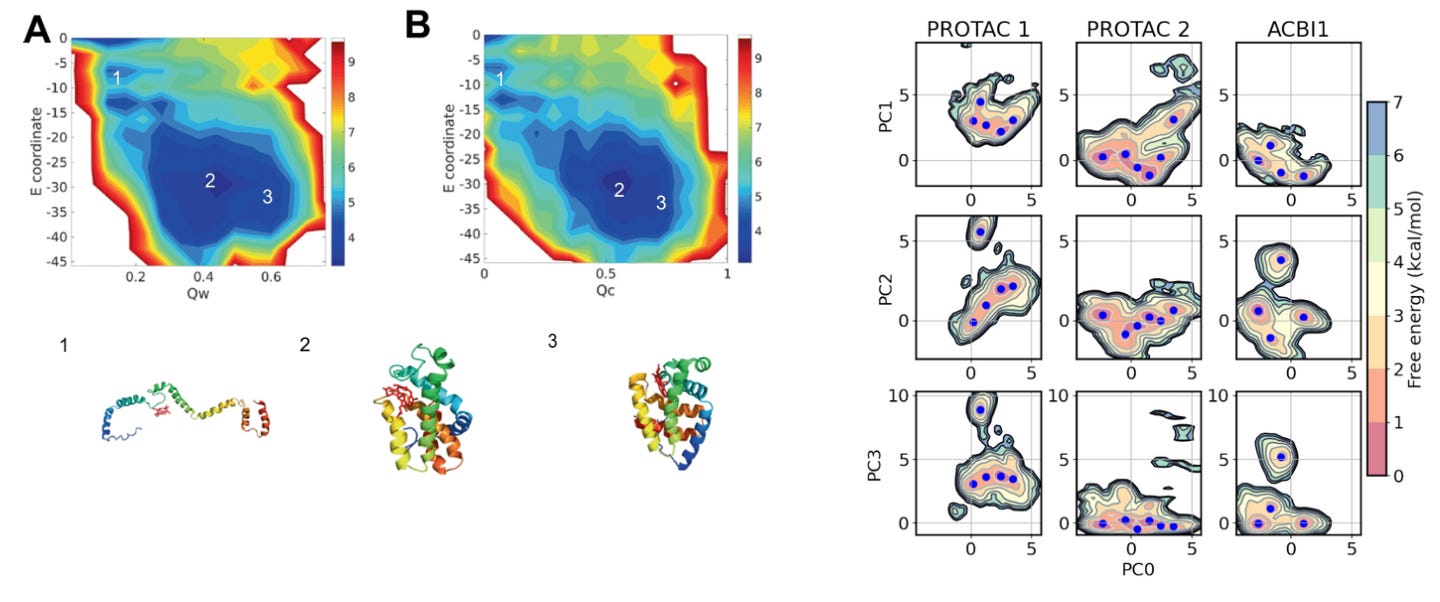
Protein complexes arising from induced proximity drugs are less consistent. Without co-evolution, induced complexes can bounce between a multitude of semi-stable forms, as shown above (right). This perplexes degrader design. In the below example, one group showed how three similar PROTACs could create nearly identical POI—E3 residue contact maps and crystal structures, yet have drastically different degradation efficiencies (DC50, DMax). How could this be?

Comparing crystal structures with MD, the researchers found that the static structures were near, but not in, global free energy minima predicting from MD. Therefore—our crystallographic gold-standard for structure-based design and ML model training isn’t always representative of biological reality. Centering on these three PROTACs’ global minima, the group simulated the full mechanism of action (MOA) for protein degradation (shown below). They found that ACBI1-bound ternary complexes adopted conformations that favorably position solvent-exposed lysine residues on the POI proximal to E2 ligase—the enzyme responsible for the catalytic transfer of ubiquitin.
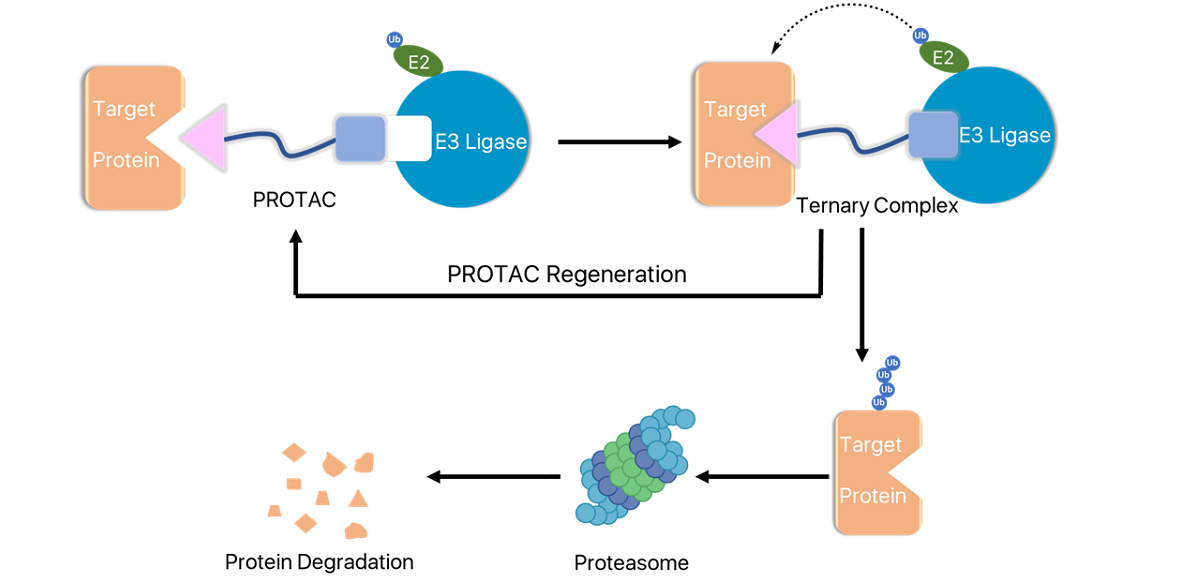
Whether a TSMD or a PROTAC—drug developers seek to selectively modulate the function of a disease-implicated target—not simply bind it. MD simulations enable scientists to model the mechanistic obstacles involved in protein degradation efficacy. These include the conformational heterogeneity of non-native protein complexes as well as the spatiotemporal proximity of solvent-exposed lysines to a cell’s supramolecular ubiquitination system.
MD-derived insights may also help drug developers enhance a PROTAC’s kinetic profile and selectivity. Optimizing lysine-E2 proximity promotes faster catalytic turnover, freeing a PROTAC up to continue degrading its target. Stabilizing protein complex conformations that expose specific lysines may help PROTACs degrade only certain members of a protein family. Altogether, MD seems especially critical for exploiting the ostensible advantages of PROTACs—many of which having generated early proof-points in the clinic. Efficacy isn’t everything, however. Drug developers must co-optimize a degrader’s efficacy alongside other characteristics like solubility, permeability, and others, that contribute to a drug’s absorption, distribution, metabolism, excretion, and toxicity (ADMET) properties.
The TSMD field has accumulated vast knowledge around what features of molecules impact ADMET properties. While these so-called molecular descriptors (e.g., topological polar surface area (TPSA)) assist in optimizing TSMDs, they fail for PROTACs. Crudely, this is because PROTACs are big and flexible. They violate the oft-cited Rule of Five (Ro5)—a historical guiding principle for making human-tolerable medicines. As such, the task of mapping the physiochemical properties of PROTACs that impact ADMET is presently a dark art. Alongside efficacy, we argue that MD could serve illuminating role here just as well.
Beginning with absorption—the first stop in the ADMET journey—things already get complicated. Several studies suggest that compounds weighing more than 1,000 daltons will have nearly zero cell permeability. Vexingly, nearly a third of PROTACs in development weigh more than this. What gives? The structural flexibility of degraders allows them to morph between hydrophobic and hydrophilic states in response to their environments. This is called chameleonicity and it’s a real word!
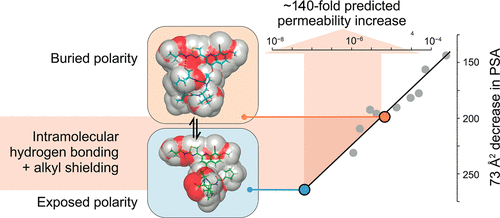
Building useful in silico ADMET predictors for PROTACs will require combining large experimental datasets with a new set of molecular descriptors. Fortunately, our permeability assay armamentarium continues to grow, including several like PAMPA, Caco-2, and CAPA. Several studies suggest that 3D (and indeed 4D) MD-derived descriptors are particularly informative for ranking permeability. From first principles, it reasons that simulated conformational trajectories could serve as rich substrates for mechanistic insight of PROTAC permeability—and indeed, other salient properties. This seems like a terrific place for groups to develop foundational datasets for the industry. After all, the largest database of PROTACs for training QSPR models has just ~3,000 entries—an order of magnitude smaller than for inhibitors where property prediction is easier, but not trivial.
Like many technologies empowering drug development, MD is a pillar of PROTAC design—not a panacea. The accuracy and rigor of MD simulations requires a tradeoff. The compute intensiveness of MD scales super-linearly with the number of atoms involved in a simulation. This prohibits its use as a virtual screening tool. Indeed, many groups employ MD with a barbell strategy—at the beginning to resolve a target, its binding pocket(s), and any relevent conformational dynamics—and in lead optimization to understand lead candidates’ efficacies. Even so, clever MD techniques, sometimes combined with ML, can help scientists squeeze the most out of their fixed compute capacity.
Enhanced sampling, for example, is a set of advanced MD techniques practitioners can use to explore the vast configurational landscape of ternary complexes more efficiently. One type of enhanced sampling is weighted ensemble (WE) analysis which involves running many parallel simulations. Trajectories that get ‘trapped’ in energy minima quickly stop becoming useful—these simulations are pruned, saving compute resources for trajectories that’re still exploring novel portions of the conformational landscape. Along these lines, practitioners can use replica-exchange (REMD) to create parallel simulations under different conditions (e.g., temperature), hopefully encouraging simulations to overcome energy barriers. In many cases, users can also select a collective variable (CV)—a problem-relevant metric (e.g., end-to-end distance) that servers to reduce the dimensionality of a simulation. Despite all these, advanced researchers have reported being able to dock just 500-1,000 PROTACs and run MD for 50 lead candidates using a combination of 64 GPUs and 500 CPUs.
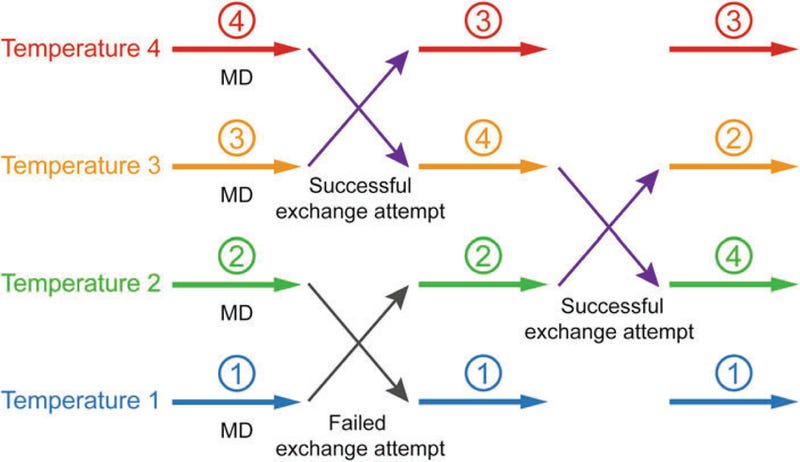
For many reasons, MD is an exceptionally informative technique for precisely deciphering the effectiveness and physiochemical properties of PROTAC molecules. No technique lives in a vacuum, however. Like all physics-informed methods, MD is rate-limited by compute resources. Data-driven (ML) methods are fast, though oftentimes less accurate, especially when considering the juxtaposition of data sparsity and problem complexity of PROTACs. As has shown to be the case, clever ensemble techniques combining structure-based and data-driven methods seem to occupy the state-of-the-art (SOTA) for drug design. MD represents just one path forward, yet there are many exciting developments, such as geometric deep learning, that are flourishing in parallel. Therefore, this shouldn’t be taken as an exaltation of MD above other methods, but rather as a spotlight on an established technique applied to a new problem space.
PROTACs face many challenges outside the scope of MD—such as issues revolving around their synthesis. Indeed, this has fueled the industry’s interest in molecular glues—smaller compounds that also induce proximity between POIs and E3 ligase. I’m interested to see if and how MD becomes relevant to each of these new areas. Nevertheless, our toolkit for attacking PROTAC design is expanding and that’s something to get excited about.
At Dimension, we’re passionate about the interface of the improbable and impossible within life sciences and technology. We’re excited about the future of MD and ML. If you’re a founder, technologist, academic, or otherwise involved in this space—please feel free to DM me!