Unnatural Amino Acids
The extended amino acid alphabet is full of strangeness, but may hold the key to breakthrough applications in the life sciences.
Introduction and Contents
Amino acids are the building blocks of proteins—one of the most common and important macromolecules on the planet. Nature has evolved a roughly 20-member library of amino acids that all organisms share. Peculiarly, scientists have catalogued over 500 rare amino acids in the wild. Think of these like a secret annex to the 20-member amino acid library or a collection of rare books. They exist. They’re important. They’re mysterious. Where do these additional amino acids come from? Why do they exist and what do they do?
I aim to tackle all of these questions and more with this essay, with a particular tilt towards the growing therapeutic utility of so-called unnatural amino acids. Folks already familiar with the basics of proteomics—amino acids through translation—can skip guilt-free through the first section. Enjoy!
Table of Contents
Basic Primer
Protein Overview
Proteins are Nature’s foot soldiers. These biomolecules can do almost anything. They catalyze biochemical reactions, facilitate DNA replication, help cells communicate with each other, transport cargo around the body, and even help us see. Antibodies are proteins. Many hormones are proteins. More and more medicines are proteins. But what makes a protein a protein?
Proteins are built up from a roughly 20-letter alphabet of building blocks called amino acids. The number, type, and order of amino acids give a protein its identify, structure, and physiochemical properties. Smaller segments of a protein are called peptides or polypeptides and they too are composed of amino acids.
Similar to linguistic alphabets, each amino acid is unique—it produces its own ‘sound’. As highlighted below, all amino acids have some parts in common. They share the same backbone structure inclusive of a carboxyl group (—COOH) and an amino group (—NH2) oriented around a central (alpha) carbon atom. Where they differ is in their R-groups, which is the lazy chemist’s way of writing ‘rest of the molecule’.
R-groups dictate the identity and physiochemical properties of an amino acid. Some R-groups are small and uncharged while others are large and charged. Some R-groups are linear while others contain cyclic structures, as shown in the table below. The important takeaway is that each R-group is unique and Nature has evolved roughly 20 that are used commonly throughout life on Earth. For anyone interested in the question of, “Why these particular 20 amino acids?”—I’d recommend reading Frozen, But No Accident.
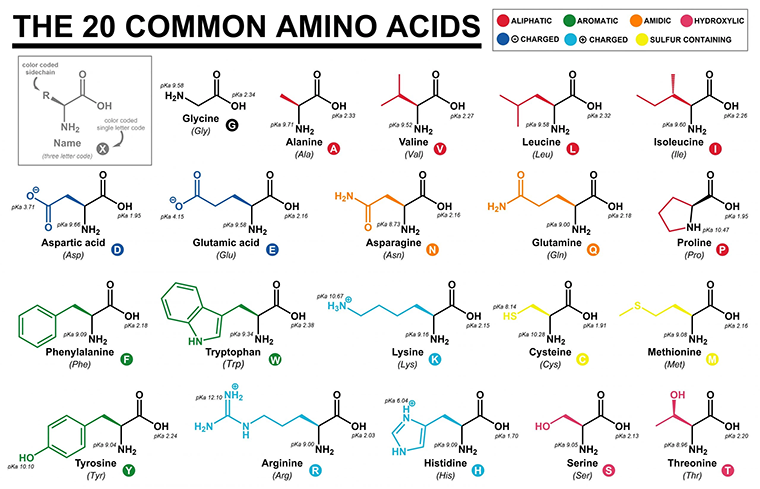
Polypeptides are linear as they’re first formed inside cells. The carboxyl group of the first acid forms a peptide bond with the amino group of the second acid whose carboxyl group forms another peptide bond with the carboxyl group of the third acid, and so on. This means that adjacent R-groups interact with one another in the first moments of the polypeptide’s lifespan, causing the flexible backbone to contort.
Adjacent, similarly charged R-groups might kink the backbone apart while adjacent, oppositely charged R-groups might bend the backbone in on itself. Before long, amino acids that were once distant in linear, sequence space are now close to one another in 3D space. These new dynamics cause the protein to bend into a stable shape (conformation) where it lives a good portion of its life, as shown in the diagram below. If you want to know more about protein folding and specifically how machine learning (ML) models like AlphaFold3 (AF3) do this computationally—check out my recent Field Guide to AF3.
A protein’s shape dictates its overall biomolecular properties. Once shuttled to its proper location in the body and surrounded by other molecules, these properties dictate the protein’s function. In that way, the role of an entire macromolecule ascends from the simple ordering of a 20-letter amino acid alphabet. But how exactly are polypeptides formed? What is the machinery that transforms a flotilla of amino acids into peptides?
Translation Overview
Amino acids are assembled into nascent proteins through a process called translation that occurs in a macromolecular machine called a ribosome. As diagrammed below, a messenger RNA (mRNA) molecule carrying the genetic blueprint for a peptide leaves the nucleus and finds its way to the small ribosomal subunit. Recall that mRNA is built up from a four-letter nucleotide alphabet containing A’s, C’s, G’s, and U’s. A long time ago, my mnemonic for translation centered on the conversion between two alphabets, the four-letter nucleotide alphabet and the 20-letter amino acid alphabet.
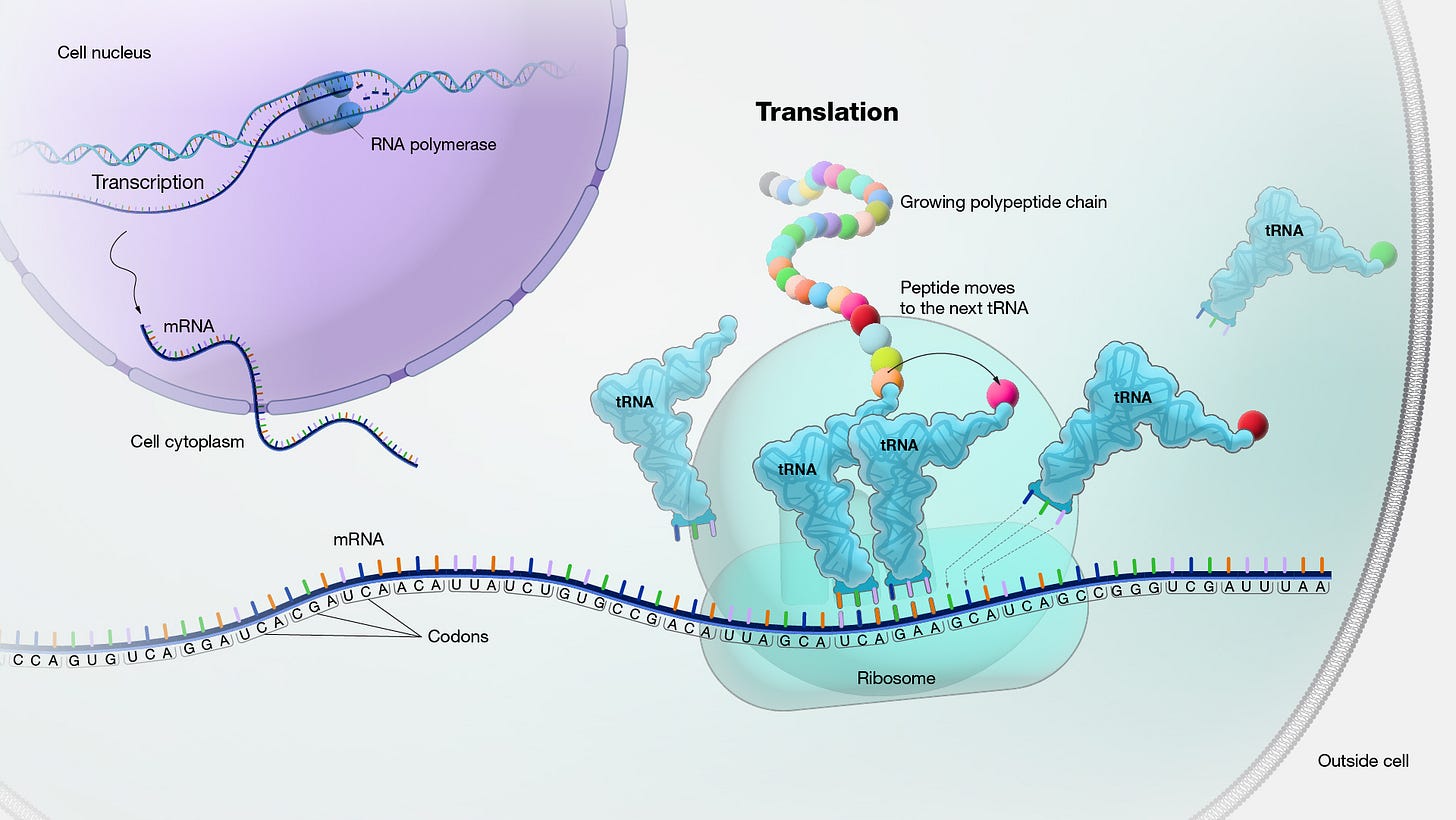
mRNA nucleotides are grouped into triplets called codons. Codons are the minimum chunks of information that map to amino acids. Importantly, this mapping isn’t 1:1 because there are 64 unique, three-letter permutations of four nucleotides. The rules governing how codon triplets are translated into amino acids follows the below codon chart. Readers may notice that only 61 codons code for amino acids while three others serve as STOP codons—signals for the translation process to end. This also implies the question of what codon STARTS the translation process.

While heuristics and charts are helpful, they’re also abstract. What I find beautiful are the physical underpinnings of these short-hand rules we use to simply the chaotic process of protein translation. By examining some of the other actors in the story, like transfer RNAs (tRNAs) and amicoacyl-tRNA synthetases (aaRSs), we gain a deeper biochemical appreciation for where our mnemonic devices come from. This level of rigor is also necessary to properly explain the main topic of this piece—unnatural amino acids.
Translation is divided into three phases: initiation, elongation, and termination. Once an mRNA molecule binds the small ribosomal subunit, a specialized molecule called tRNA begins to scan the mRNA for the codon AUG. tRNA molecules are like little tugboats. They each carry a single amino acid from the cellular milieu to the physical site of translation.
In this context, scanning means that the tRNA is running its bottom portion—called an anticodon—along the mRNA until it finds a START codon (AUG). Once bound and anchored, the large ribosomal subunit attaches, forming a full ribosomal complex and ending the initiation phase. Ribosomes have three 'grooves’ that tRNAs fit into during the elongation phase: E (exit), P (peptidyl), and A (aminoacyl), as shown below.
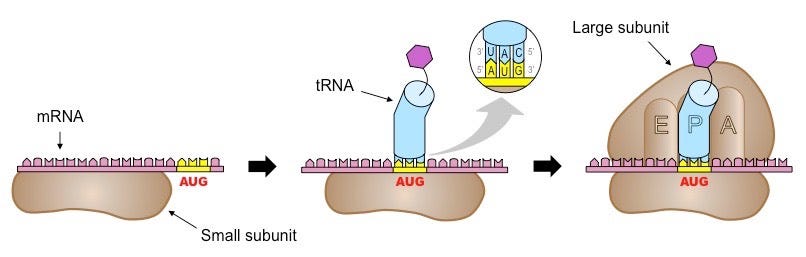
Thus begins the cyclic elongation phase. Here, the next codon following AUG is recognized by its cognate tRNA. Enzymes facilitate a peptide bond between the carboxyl group of one amino acid with the amino group of its adjacent amino acid. The nascent peptide extends at the A site and the complex ratchets rightward along the mRNA’s length. The amino acid-less tRNA is jettisoned at the E site and the process begins anew.
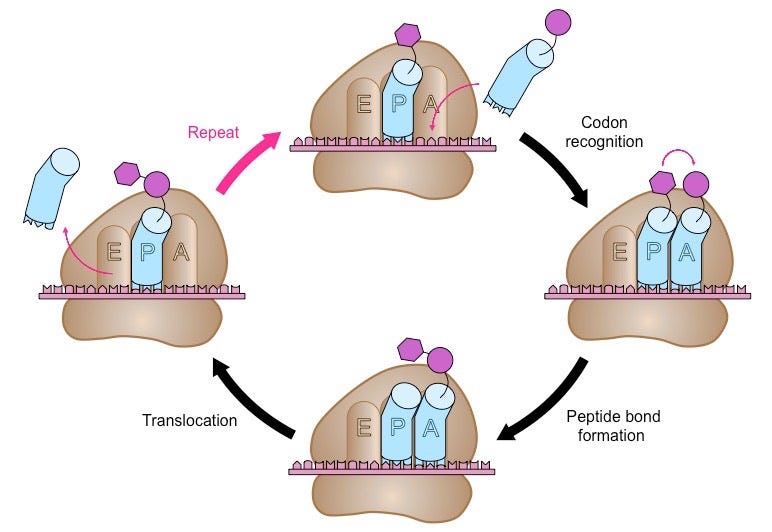
Termination happens when one of three STOP codons summons a release factor, which breaks apart the entire complex and frees the polypeptide to begin folding into whatever shape its amino acids dictate, as shown below. To give a sense of scale, protein translation occurs at a rate of 10 amino acids per second, meaning it would take about a minute to synthesize a hemoglobin protein.
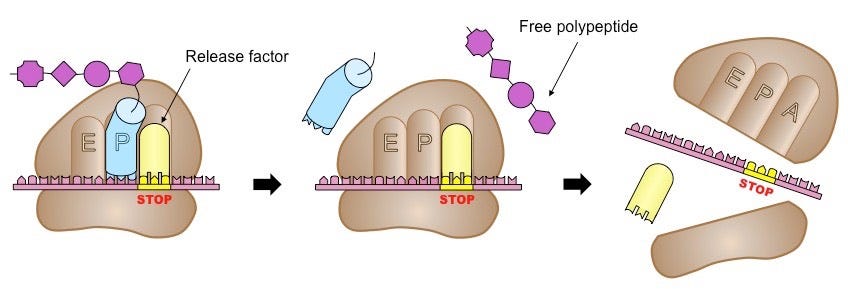
uAAs and Their Applications
Unnatural Amino Acid Overview
Across all kingdoms of life on Earth, there are very few exceptions to protein translation occurring outside the codon rules we’ve established. Genetic recoding, the idea that amino acids can be added through non-normal codons, does seldom occur context-specifically in some fungal and bacterial species. There is a vast and strange world, however, of unnatural amino acids. This extended alphabet doesn’t leverage genetic recoding—in fact, it doesn’t rely on genetic encoding at all.
Unnatural amino acids (uAAs) harbor similar backbones to their natural counterparts. uAAs have both carboxyl and amino terminal groups. Where they differ is their R-groups. uAAs feature a novel set of R-groups, some that diverge slightly from natural R-groups and others that look and act quite alien.
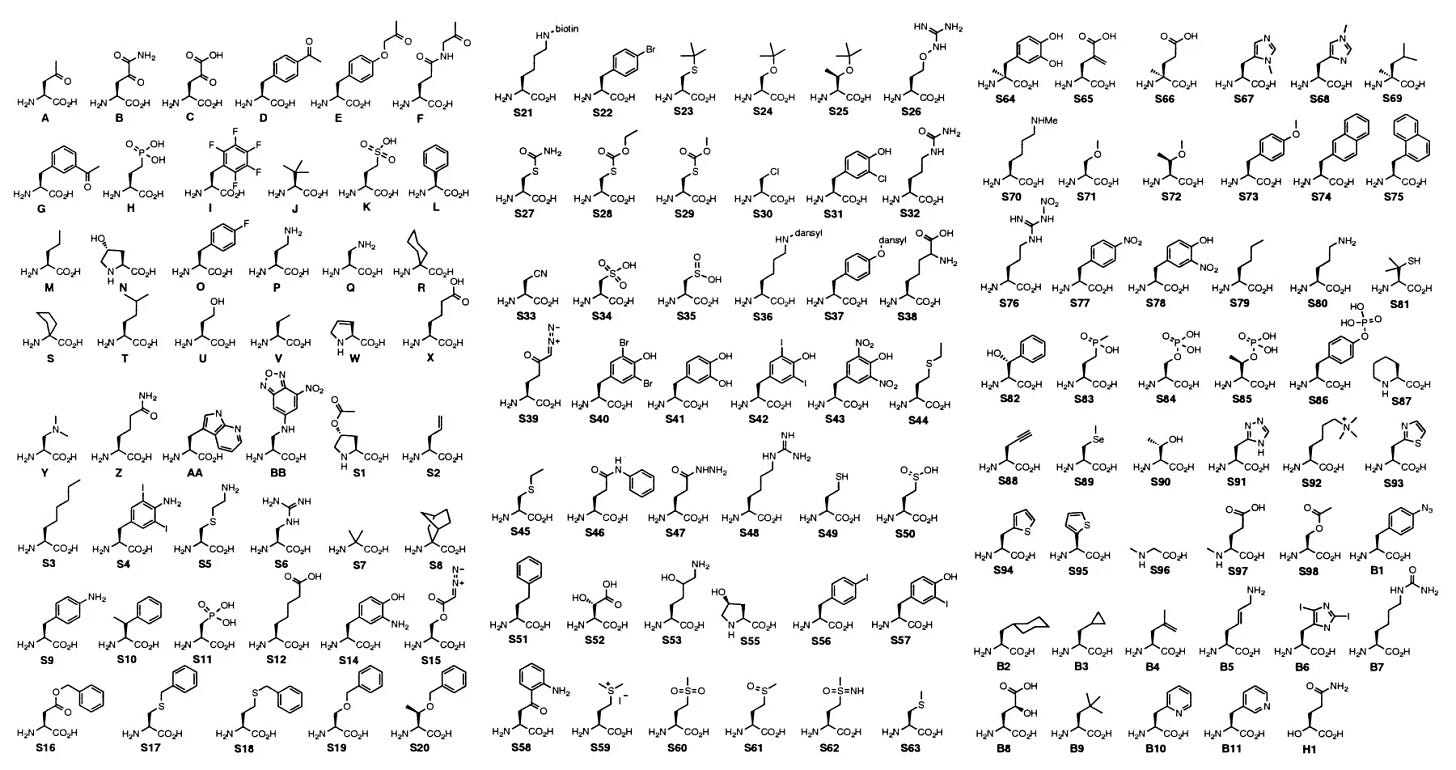
uAAs aren’t directly coded for using the codon table. Instead they exist as precursors, analogs, or metabolic intermediates of natural amino acids. Nature has also evolved a separate set of enzyme complexes capable of incorporating uAAs within nascent peptides. For example, polyketide synthase (PKS) and non-ribosomal peptide synthetase (NRPS) can create proteins harboring uAAs, though these reactions are very bespoke.
Despite the name, uAAs are found throughout plants, animals, and microorganisms. uAA-containing peptides are functionally diverse. Plant uAAs are often produced in response to threats. Some species of grass release L-m-tyrosine from their roots which acts as a toxin. Bacterial and fungal uAAs are commonly known for their antibiotic and anti-inflammatory properties. While rarer, animal uAAs are generally metabolic intermediates of canonical amino acids and are used clinically to reverse liver damage and as diagnostic biomarkers. Altogether, researchers have discovered >500 uAAs.
uAA Applications
Since the discovery of uAAs, scientists have sought to engineer them into peptides. After all, if Nature has found uses for them, why shouldn’t we? The first in vitro, site-specific incorporation of a uAA into a peptide was reported in 1983. In 1997, the first genomic encoding of a uAA was executed in vivo. Exactly how these feats were accomplished is something we’ll discuss later.
The applications of uAA-containing peptides are as diverse as uAAs themselves. From a basic research perspective, scientists can introduce fluorescent/photo-crosslinkable probes into peptides at specific sites using uAAs. This allows for tracking of protein dynamics and protein-protein or protein-ligand interactions. Scientists can also mimic post-translational modifications (PTMs) with uAAs, facilitating our understanding of endogenous PTMs and their functions. Photo-caged uAAs can allow for light-activated control of protein function, enabling spatiotemporal studies of protein activity in cellular contexts. Despite all these, therapeutic protein engineering is the fastest growing application area for uAAs.
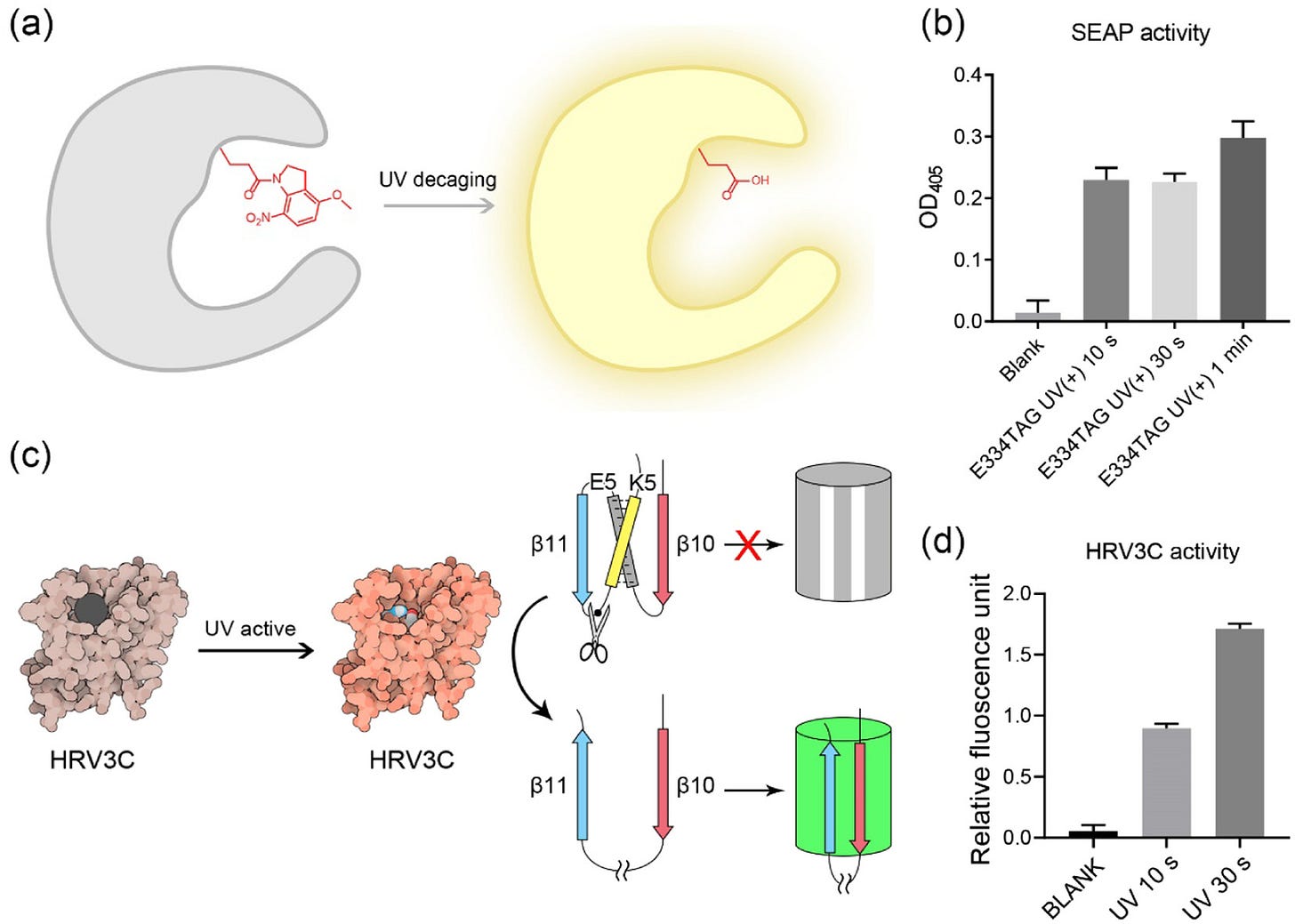
Drug Conjugates
Conjugate therapies are entering their golden era. Conjugates are a class of drug where a therapeutic payload is engrafted onto a targeting agent. Ostensibly, the targeting agent enables greater site-specific delivery of the payload compared to if that payload was delivered by itself into the bloodstream. Antibody drug conjugates (ADCs) are perhaps the most well known example of a conjugate therapy. Radioligand therapies (RLTs) are another. I’ve written about both in previous blogs, which you’re free to read here and here, respectively.
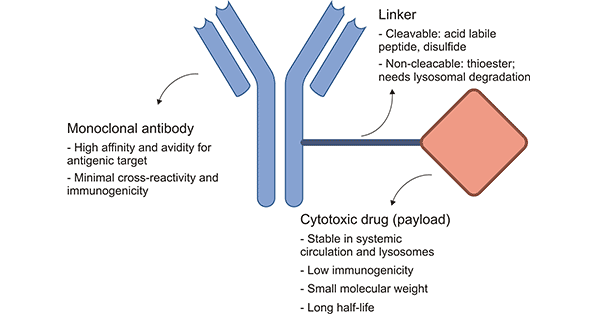
Most targeting agents are peptide-based. Therefore, drug developers must use a technique called bioconjugation to attach the therapeutic payload to an amino acid. Chemists generally exploit solvent-exposed lysine or cysteine amino acid residues for bioconjugation.
Lysine contains an amine group while cysteine contains a thiol group—both of which are highly nucleophilic, meaning they’re reactive under mild conditions. This makes lysine and cysteine ideal residues for bioconjugation chemistries. This technique has given rise to the first wave of FDA-approved conjugate drugs.
Unfortunately, drug developers can’t always choose exactly where or how many lysine or cysteine residues are present on the targeting agent. If too many or too few payloads glom onto the protein or if they attach to different residues, it results in a heterogenous drug product pool. This requires expensive downstream purification, lowering product yield and resulting in higher prices.
Drug designers can encode uAAs into targeting agents to overcome the issue with heterogenous product manufacture. By leveraging the diversity of R-group chemistries and the fact that uAAs don’t naturally appear in antibodies or small peptide binders, it allows chemists to selectively attach payloads precisely where they wish and drive those reactions to completion. Furthermore, this enables fine-tuning over these drugs’ pharmacokinetic (PK) profiles and increases the likelihood of homogenous product pools.
Site-specific incorporation of payload or other prosthetic groups is just one therapeutic application of uAAs. Perhaps an even more impactful area, and one that still resembles the Wild West, is protein medicinal chemistry.
Protein Medicinal Chemistry
Lead optimization is a staple of both small molecule and antibody drug development. During this development phase, scientists make minute refinements to small molecule or antibody scaffolds to improve their physiochemical properties. This process can be likened to multiparameter optimization as drug developers juggle changes that could improve solubility, but worsen potency, for example. Lead optimization for peptides is much less mature by comparison.
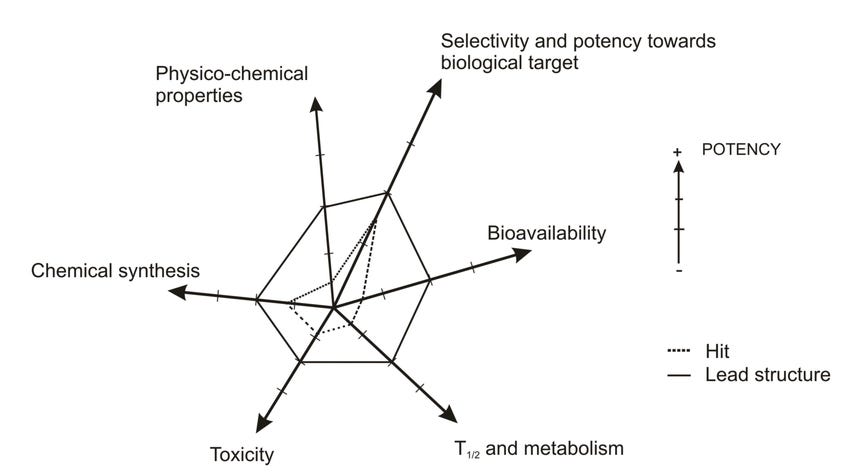
Protein biochemists may begin lead optimization by replacing each amino acid in a peptide with alanine—a simple amino acid. This is known as alanine scanning and it helps researchers understand the functional impact of each amino acid in the sequence, giving insight as to where and how further modification might affect bioactivity.
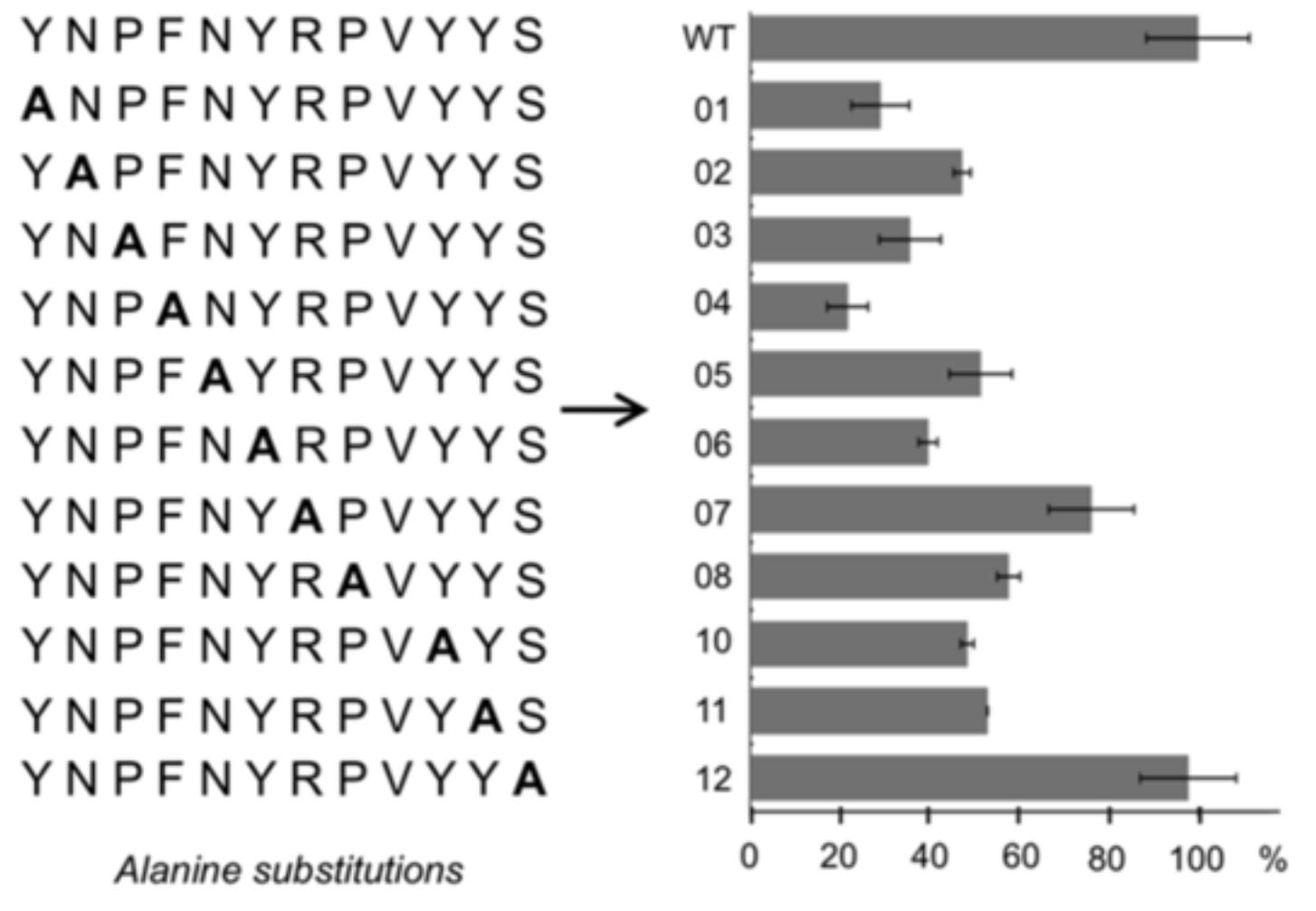
Drug developers first modify peptide backbones. For example, biochemists can add structural rigidity and protect against enzymatic degradation all by bending the peptide’s backbone back onto itself—a process called cyclization. Developers can make large changes to binding surfaces by installing defined secondary structures like alpha-helices or beta-pleated-sheets. Unsurprisingly, uAAs have a major role in protein medicinal chemistry just as well.
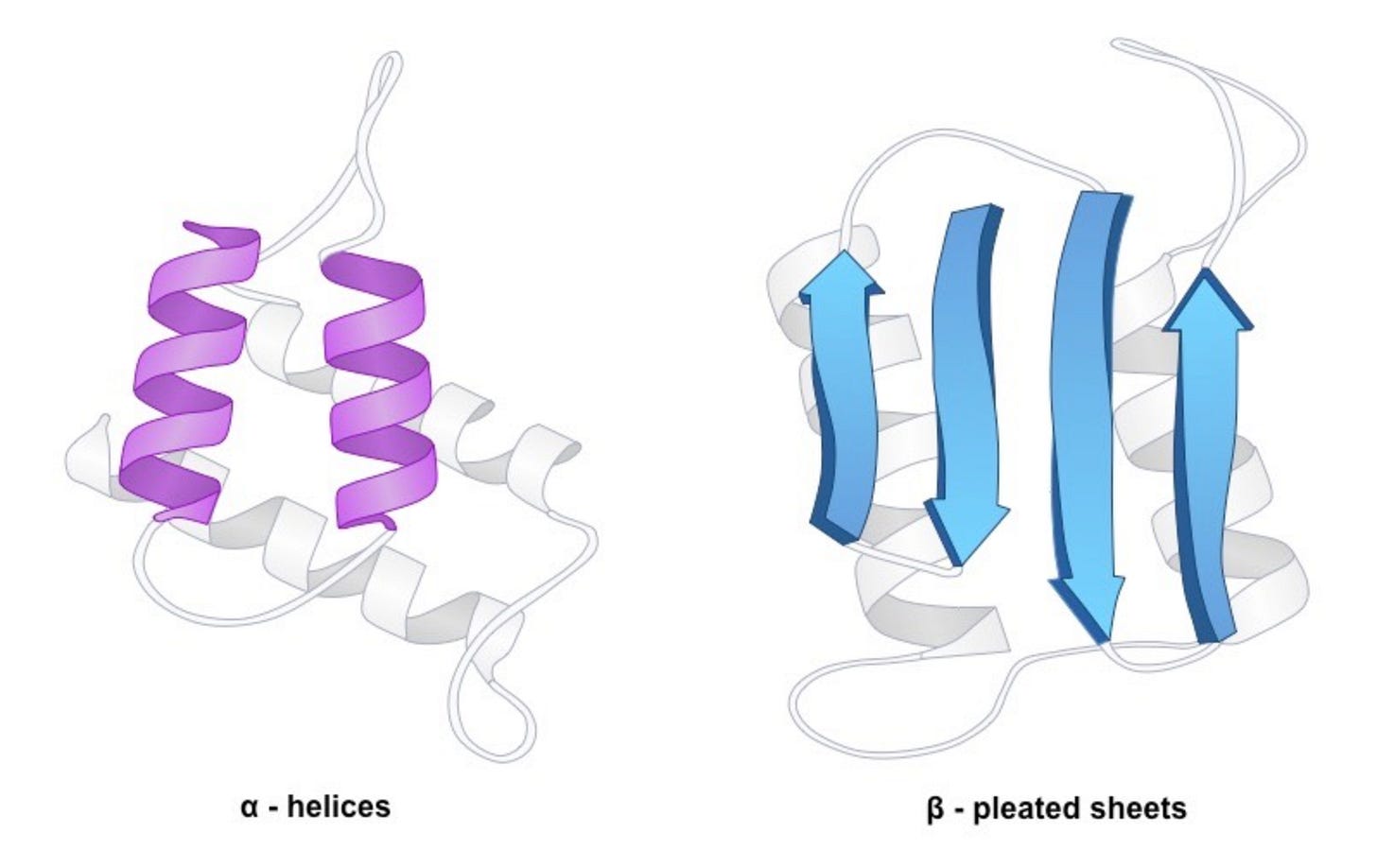
The world of peptide uAA modifications is extraordinarily vast. Scientists have looked towards natural uAA-containing proteins like cyclosporin A, tryglysin A, and darobactin A, to understand uAA-specific structure-activity relationships. Indeed, several classes of uAA seem to confer increased resistance to proteolysis, increased potency, cell permeability, and receptor selectivity, amongst others.
These lessons have been leveraged prospectively by drug developers. Two examples include Chugai’s RAS inhibitor (LUNA18) and Merck’s PCSK9 inhibitor (MK-0616). These macrocyclic compounds contain four unique uAA modification classes to enable greater cell permeability and oral bioavailability, respectively.
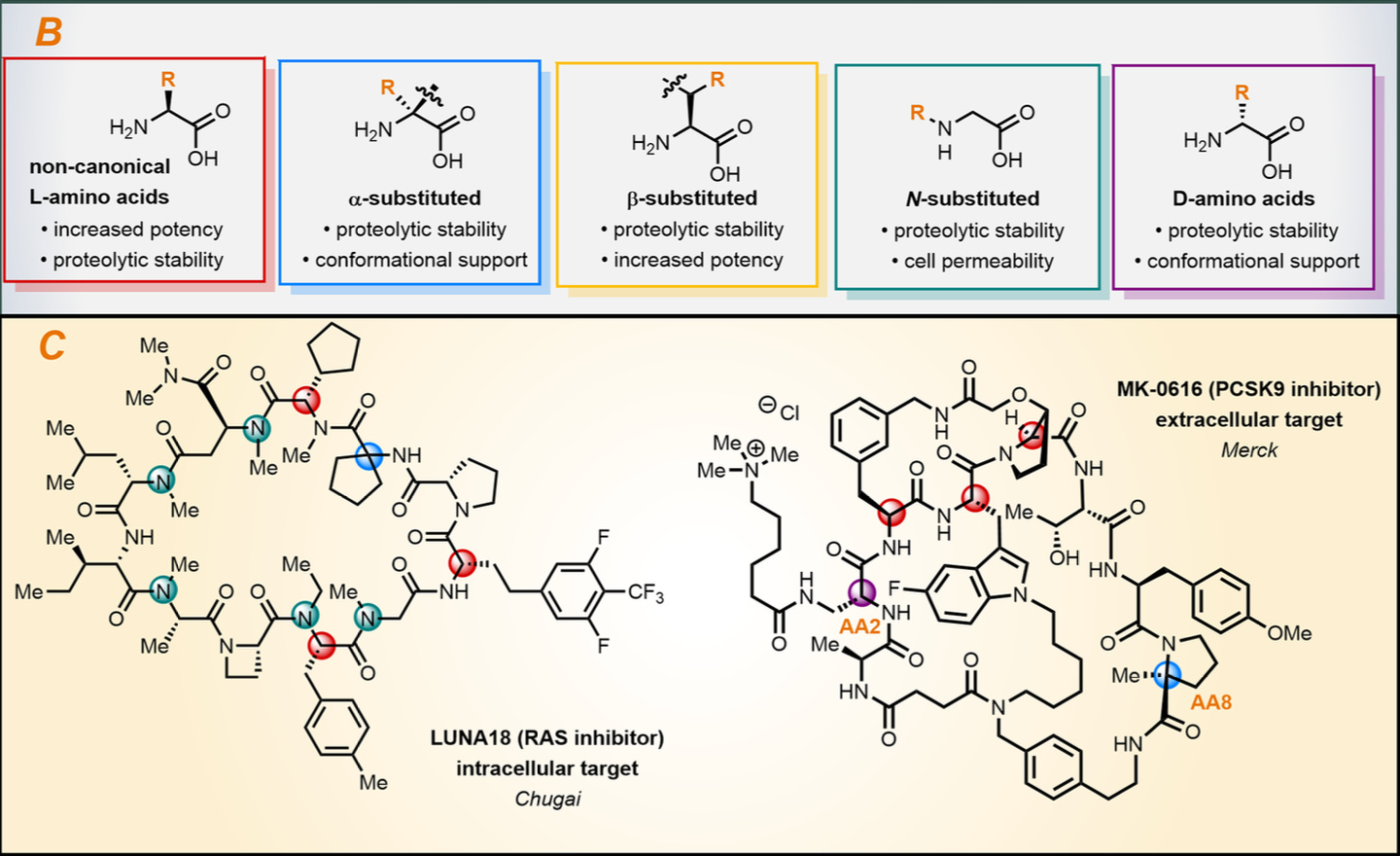
Peptide therapeutics, a historically maligned category of drug, is having a renaissance. Accounting for 5% of global pharmaceutical sales, peptides are a $70B market. Just 34 peptide drugs were approved from 1960-1999 while 52 have been approved this century, a rate tripling from 0.9 approvals per year to 2.7 approvals per year. Biologics, including antibodies, are a monster category—accounting for 20% of the global pharmaceutical industry. The combination of quickly growing protein and peptide-based modalities and the increasing utilization of uAAs in biologics suggests that uAAs will play a critical role in the present-future of the pharmaceutical industry.
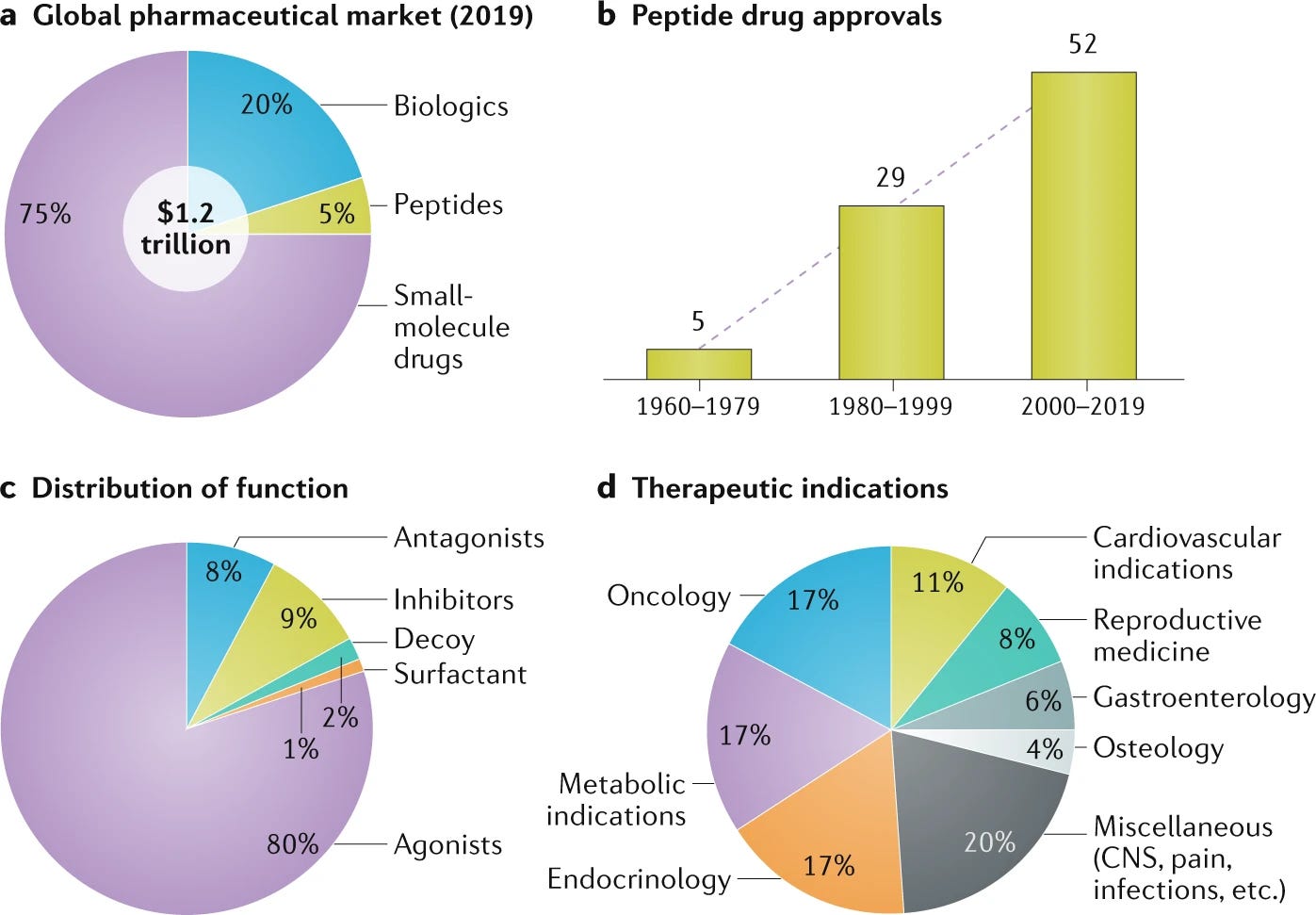
Frontiers of Work
This final section deals with areas of active research furthering what’s possible with uAAs and uAA-containing peptides. These topics range from chemistry to biology to machine learning and everything in between. One main takeaway is the non-virtuous cycle between data generation and machine learning in the uAA-adjacent sciences.
uAA-containing peptides are hard to make, which means training data is scarce, which means models are less performative, which means industrial applications are less tractable, which means there’s less demand pull to make uAA-containing peptides easier to make, and so on and so forth.
Synthesis of uAAs
uAAs need to be synthesized before they can be installed into proteins. Scientists have a variety of methods to create uAAs—each with their own advantages and disadvantages. Oftentimes, the challenges with uAA synthesis drives researchers to purchases uAA from reagent suppliers rather than embarking on the synthesis journey themselves. Nevertheless, both suppliers and academics alike are pushing the frontier of how we make uAAs.
Broadly speaking, one can choose between purely chemical synthesis, chemoenzymatic synthesis, and cell-based, metabolic synthesis.
Purely chemical synthesis involves traditional organic chemistry mechanisms spread across multiple reaction steps and aided by chemical catalysts. This method is generally scalable and more deterministic than biosynthesis. Diverse uAA structures are accessible with chemical synthesis. However, this method involves harsh reaction conditions and has the potential to be inefficient and costly. Given the precise 3D geometries of uAAs, chemists use temporary protective groups and chiral auxiliaries to control against incorrect stereochemical intermediates. These chemical groups add to cost and decrease product yield as the number of reaction steps grows exponentially.
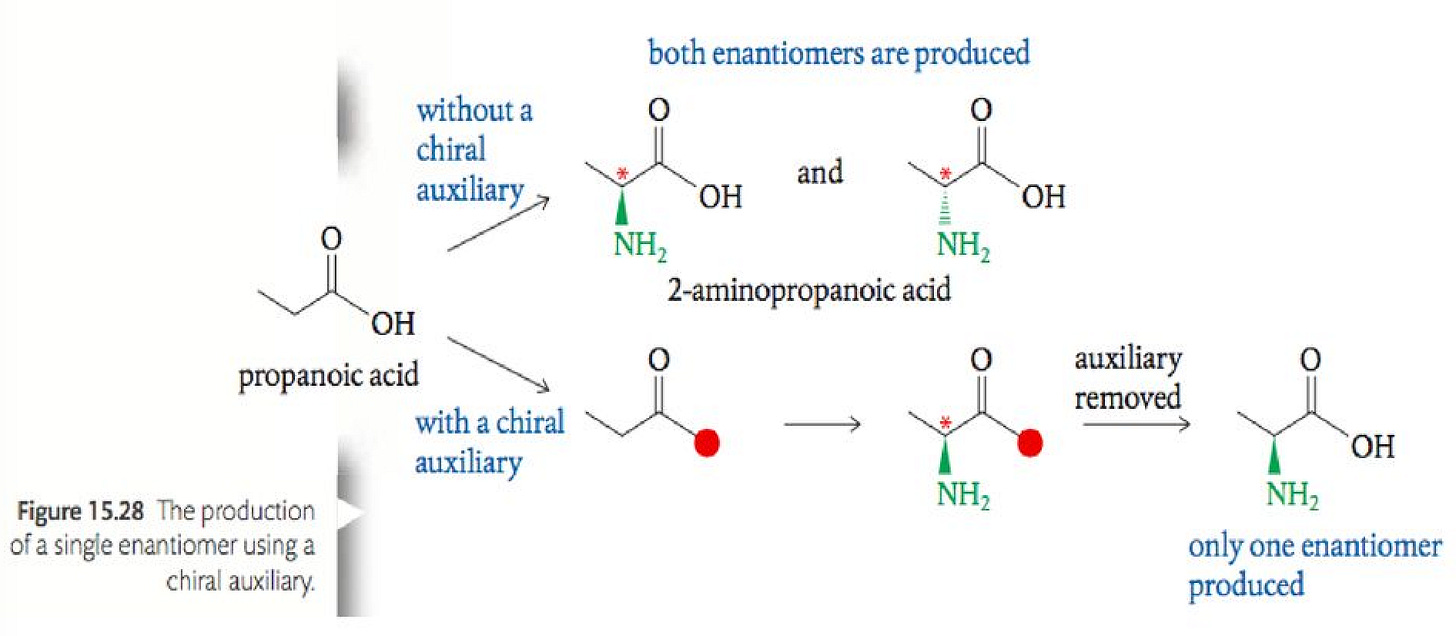
Chemoenzymatic synthesis combines chemical and biological reagents to achieve uAA biosynthesis in a cell-free context. Compared to purely chemical synthesis, this method isn’t as wasteful, involves milder reaction conditions, and can be more stereoselective given the specificity of enzymes. This eliminates the need for transient protective groups. However, chemoenzymatic synthesis can be limited in what uAAs can be created—a downside of the specificities of most enzymes. Since enzymes are involved, process scalability becomes less straightforward.
Metabolic synthesis involves genetically engineering cells to produce uAAs. We know very little of natural uAA biosynthetic pathways, precluding our ability to easily mimic them. Metabolic synthesis can access many uAA varieties and is cost-effective at scale. Similar to other biosynthetic methods, metabolic approaches require process development and scape-up, a challenging and capital intensive undertaking. As we’ll discuss soon, metabolic synthesis offers the tantalizing possibility to synthesize and incorporate uAAs into peptides all within the context of a single cell.
Incorporation of uAAs Into Nascent Peptides
Once synthesized, protein chemists must install uAAs into peptides. Similar to the upstream synthetic process, scientists have a variety of methods at their disposal to incorporate uAAs—once again, each with their own pros and cons. These include solid-phase protein synthesis (SPPS) and recombinantly using genetic code expansion (GCE).
SPPS isn’t dissimilar from oligonucleotide synthesis. Here, standard or unnatural amino acids are flowed onto a reaction substrate one at a time. Once the reaction has terminated and the peptide has grown by one unit, the process is repeated with the next amino acid until the peptide is complete. SPPS is ubiquitous, but severely limited with peptides beyond 50-70 amino acids in length. Each step has an efficiency penalty, which grows exponentially with peptide length—making long peptides exorbitantly expensive or impossible to make. Moreover, high concentrations of amino acids are required to drive synthesis, which adds to total cost particularly when many uAAs are involved.
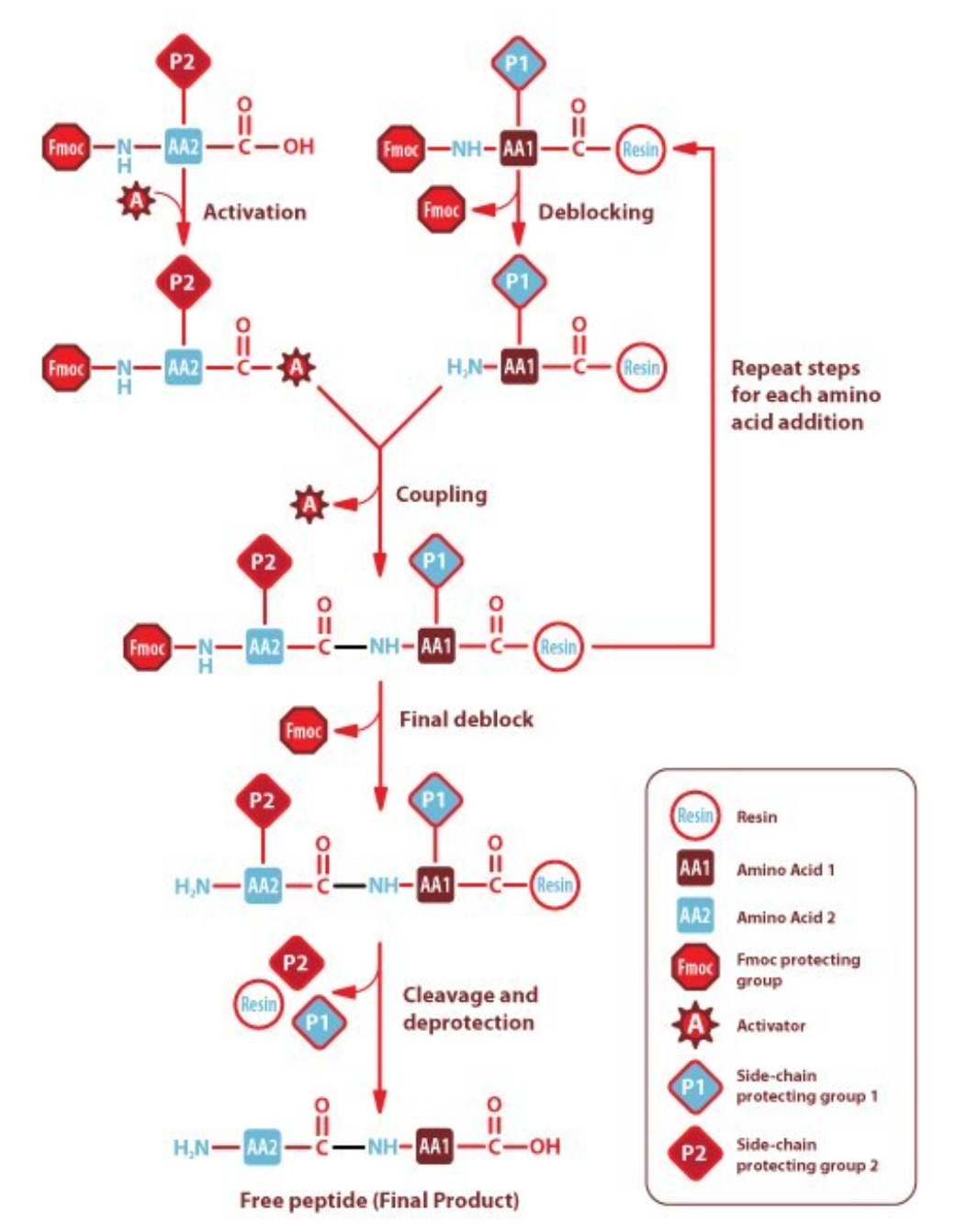
GCE is an elegant and involved process. It requires engineering a cell’s translation machinery to install a uAA at a specific site in a peptide, generally by suppressing STOP codons and reassigning them to a custom uAA-specific tRNA molecule. This ensemble of cellular mechanisms is called an orthogonal translation system (OTS) and it’s one of the most fascinating areas of uAA scientific advancement.
The main complexity arises from the fact that a cell’s endogenous translation machinery and the OTS must work simultaneously without interfering with each other. Another issue is that engineered translation systems are seldom as efficient as wild-type (WT) systems.
There’s an appreciable charge to make OTSs more catalytically efficient and versatile towards a wider range of uAA types. One body of work I find interesting is the utilization of ‘cold-evolved’ translation machinery. The hypothesis is that enzymes evolved in cold climates (psychrophilic) have high efficiencies in moderate or ambient temperatures. One research group recently tested this theory, finding that psychrophilic enzymes can be uAA-specific while also exhibiting abnormally high incorporation efficiency, as shown below.
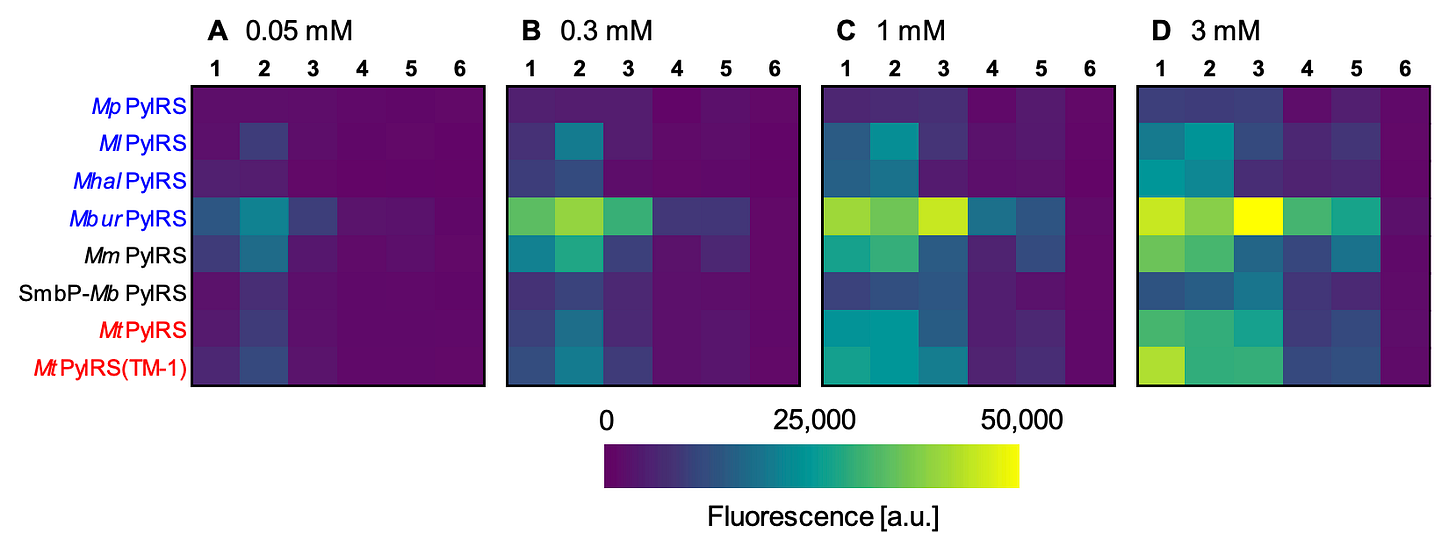
That this system can install uAAs at such low concentrations is a boon for single-cell uAA synthesis and incorporation regimes. Metabolic engineering of cells to produce uAAs is possible, but the cytosolic concentrations tend to be quite low, making incorporation with standard OTSs challenging. This could help remedy the issue.
Machine Learning Meets uAAs
Machine learning (ML) methods have supercharged protein engineering. We’ve written a review showcasing the cutting edge of computational protein engineering in the context of therapies and industrial enzymes. Proteins containing uAAs, however, pose problems for ML.
ML algorithms are only as good as the data they’re trained on. Peptides containing uAAs are underrepresented in Nature as well as experimental datasets for ML training. Therefore, models trained on standard peptides may perform poorly on uAA-containing peptides.
One unique challenge for ML on uAA-containing peptides is representation, which refers to how a model sees its training data. Peptides are mesoscale. They’re in no-man’s land. They’re not as big as large proteins (e.g., antibodies) nor as small as small molecule drugs. We typically represent large proteins using amino acids and a data format called FASTA. We represent small molecules with atoms in a format called SMILES. What should we use for uAA-containing peptides?
FASTA isn’t built to handle the expanded uAA alphabet. SMILES don’t scale well for the number of atoms in peptides. A flexible format for biomolecules called HELM has gained some traction for uAA-containing peptides, but it’s grossly unpopular compared to SMILES and FASTA. There’s no clean answer to this problem yet. Representations for uAA-containing peptides are still a mysterious frontier.
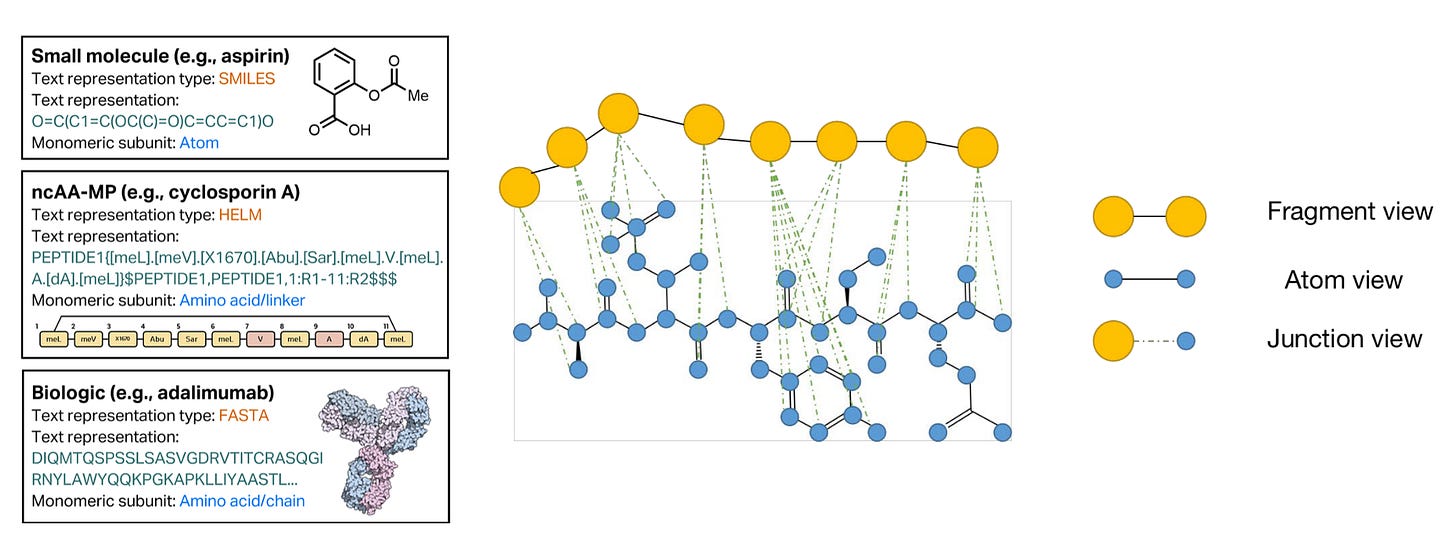
One research group concocted a multi-scale, fragment approach to uAA-containing peptide representation. This methodology uses large fragment blocks for the peptide backbone that fluidly branch off into atomic detail for side chains. Both the fragments and atoms get embedded in a heterogenous graph architecture, like the one above, for downstream prediction tasks. Amongst other uAA-specific ML accommodations, this group’s method showed improved predictive performance relative to other models across a series of tasks like binding affinity prediction and cell permeability prediction, as highlighted in the plots below.
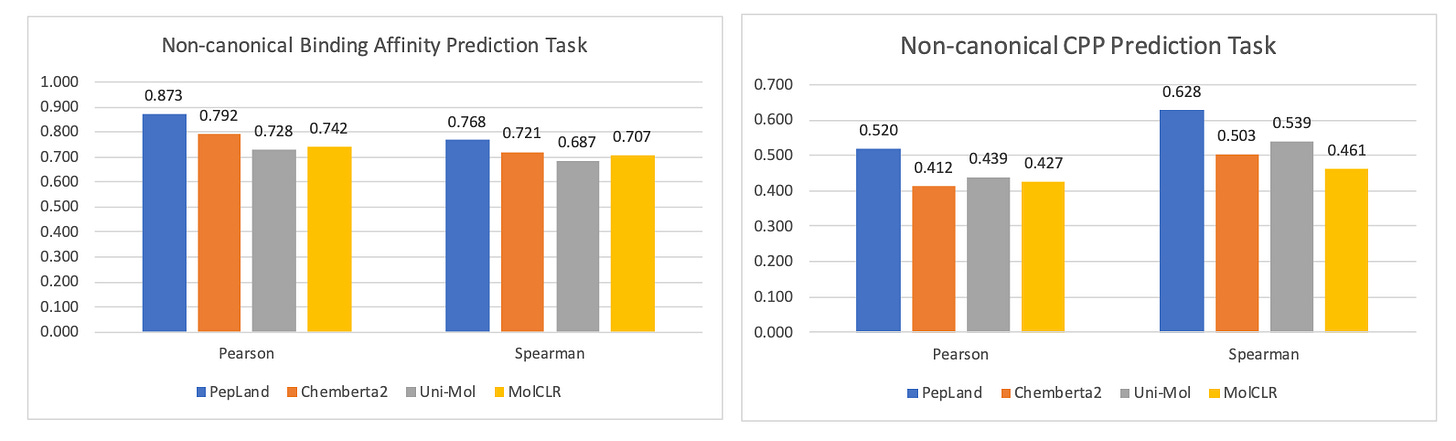
Peptide viability is a key prerequisite for higher-level tasks like property prediction or de novo design. Which sites in an arbitrary protein can tolerate uAA substitution? How will uAAs affect protein yield? Without answers to these, property prediction and design just aren’t practical. Several research groups have taken aim at this root level task, though the field is still nascent.
In 2021, one research group combined molecular dynamics (MD) simulations with ML to generate proof-of-concept learnability for how one specific uAA (acridonylalanine, Acd) might affect a protein’s viability.
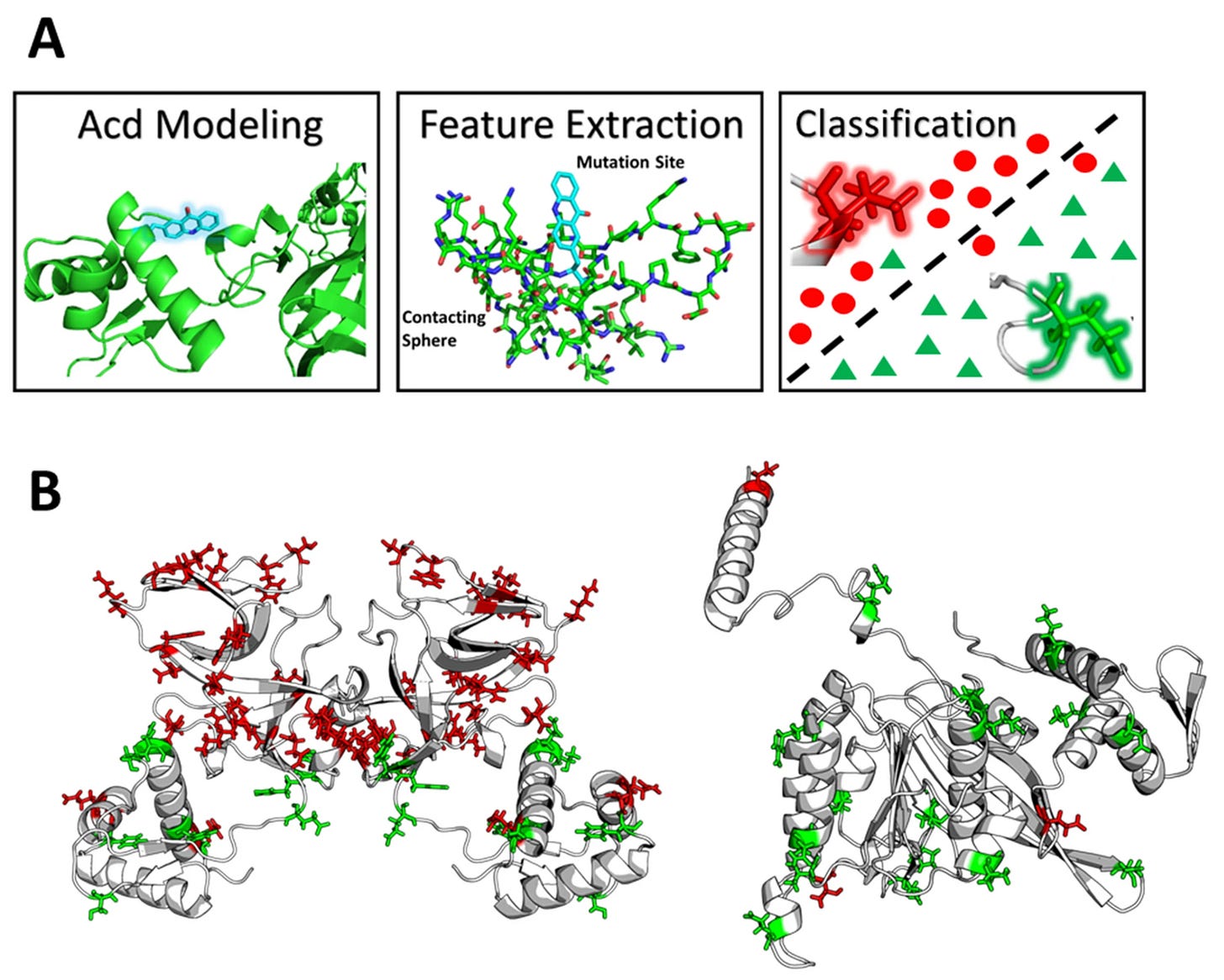
The group simulated uAA substitution into 51 unique sites across two proteins using MD. They used these MD simulations to train an ML model in lieu of physical, experimental data. The model’s goal was to predict how uAA substitution might be tolerated by these proteins—specifically, how uAAs might affect soluble protein yield. The researchers found that Acd imparted local physiochemical and steric effects that were highly predictive of site tolerability. While the model was 80-90% accurate, these results were obtained on just 10 held-out datapoints. This effort certainly isn’t generalizable to the grand problem of uAA x ML, but stands as a valiant proof-of-concept study.
Building on this effort, a second research grouped aspired to develop an ML algorithm capable of predicting site-tolerability of any uAA at any protein site. They curated a literature database of ~1,000 successful and ~200 failed uAA incorporations, each with a matching structure in the Protein Data Bank (PDB). The model considered features like changes in evolutionary entropy, steric effects, and physiochemical alterations. By examining feature importance, the second group replicated the previous hypothesis that local, uAA-induced physiochemical changes are more predictive of site-tolerability.
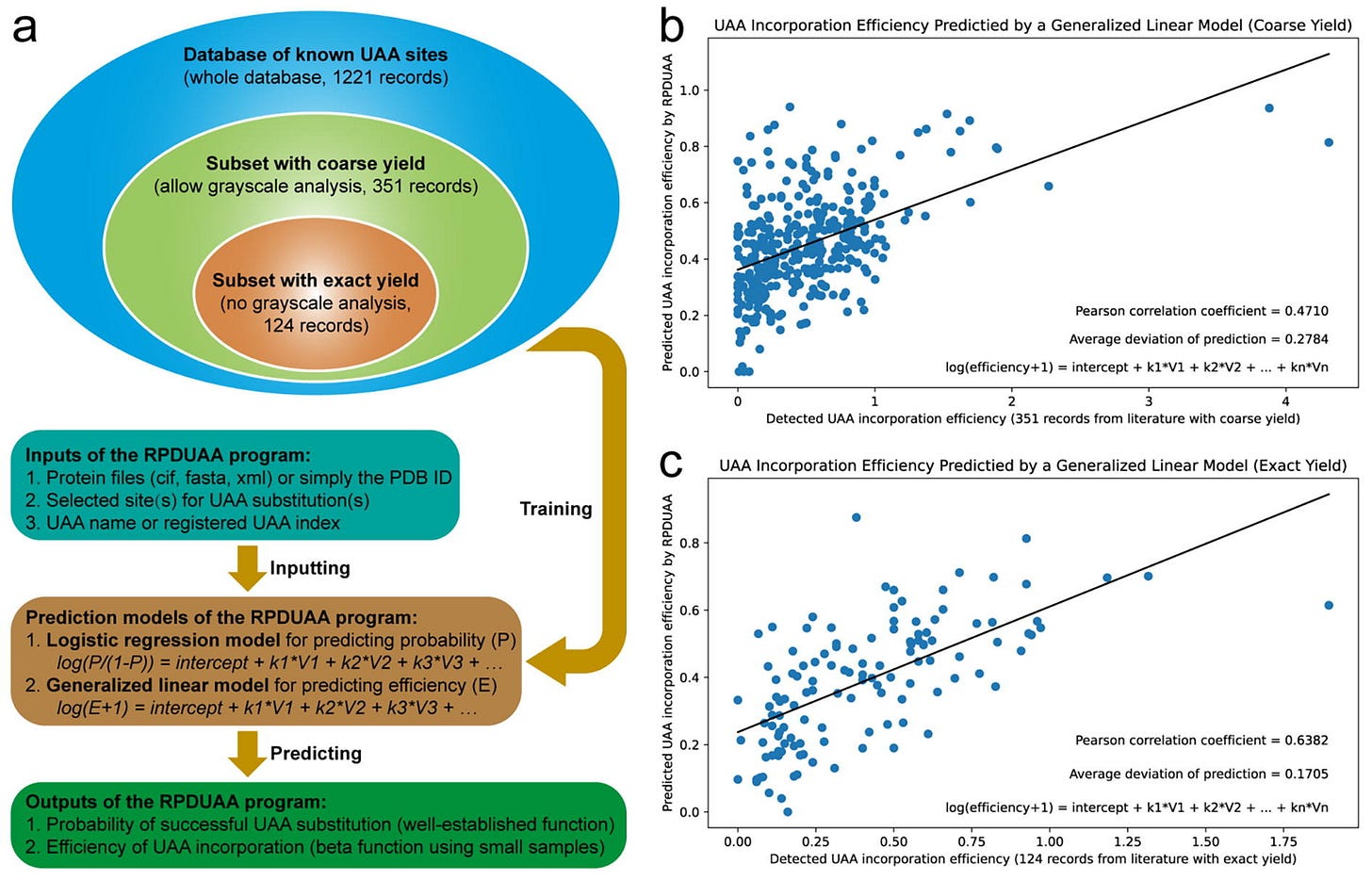
The researchers observed a decent predictive correlation to the literature (Pearson = 0.64) using the strictest training data cutoff. This is impressive given the diversity of uAAs and proteins. However, similar to the first proof-of-concept study, I suspect this model may not generalize well prospectively given (a) the lack of backbone structural diversity in the training set, (b) the fact that only one class of uAAs was used, and (c) that the training set is small on an absolute basis. Nevertheless, this work represents another critical step towards viable ML models applied to protein engineering with uAAs.
Outro
Unnatural amino acids are ancient. They’ve persisted for eons despite scant natural machinery to support their more frequent installation inside proteins. They’re functionally diverse and useful to the organisms that express them. Despite all of this, we know very little about them.
Biologics and peptide-based therapeutics are exploding in importance therapeutically and I see no reason this trend should abate. The biologics that feature uAAs seem to exhibit startlingly useful properties such as proteolytic stability. For this reason alone, my sense is more drugs will incorporate them in the future.
Whether it’s a physics-based breakthrough that enables rational uAA lead optimization despite data sparsity or a novel synthesis method that allows researchers to throttle up uAA design throughput, the design-build-test-learn (DMTA) loop of uAAs isn’t poised to stay anemic forever.
Once this flywheel starts to kick off and we begin to unravel the rules of uAAs, we will begin to wield them with more authority. This is tremendously exciting. We are tracking developments on all fronts. If you or a colleague are working on uAAs in any capacity, feel free to drop me a line on Twitter / X.
love the overview. a few misc. thoughts about the uAA and peptide tx space:
(1) lots of innovation happening in the chemical synthesis of long peptide sequences: Brad Pentelute et al have done amazing work here, see https://www.science.org/doi/10.1126/science.abb2491 for a sample of this work. there's a lot of tough engineering work that they've done to make this more effective. chemical synthesis is ultimately the way that will be most flexible here, i think, because you don't have to worry about all the issues w/ artificial tRNAs, amber suppression, etc.... particularly for peptides (and not full proteins), chemical approaches feel like the future here.
(2) particularly for macrocycles, uAAs give exquisite control over conformation, which is incredibly powerful. macrocycles are a really interesting class of peptide therapeutics, in my opinion (and lots of other people's opinions too) - enough TPSA to hit PPIs or other unconventional binding sites, but the option to still have cell permeability. i like Andre Yudin's work in this area: https://www.nature.com/articles/s41557-020-00620-y, https://onlinelibrary.wiley.com/doi/abs/10.1002/anie.202206866. also lots of work written about the "chameleonicity" of conformation-changing macrocyclic peptides, e.g. https://chemistry-europe.onlinelibrary.wiley.com/doi/full/10.1002/chem.201905599
(3) the notes about the difficulty of molecular representation are spot-on - macrocycles exist in some liminal zone where a lot of small molecule-focused computational techniques don't scale well at all, but where the assumptions of protein-specific tools are just wrong. this is true both in ML/representation contexts and simulation/modeling contexts... even just predicting what shape a macrocyclic peptide will have is brutally hard, see Yu-Shan Lin's work here (https://pubs.acs.org/doi/full/10.1021/acs.jpcb.4c00157 and others).
Fascinating science and excellent job on the post! I read that AF3 uses a mix of atomic and molecular scale tokenization. Also its a mix of transformer and diffusion models. My understanding is that transformers are limited by vocab size but diffusion isn't. In future models will we need an extensive vocabulary for each uAA and does that limit the scale we can use transformer architectures to predict uAA properties? Alternatively there are mechanistic models we could use to discover the general features across uAAs. I could imagine how this might help define the essential vocab by identifying uAAs with redundant effects on the model.